Catalogue Search | MBRL
نتائج البحث
MBRLSearchResults
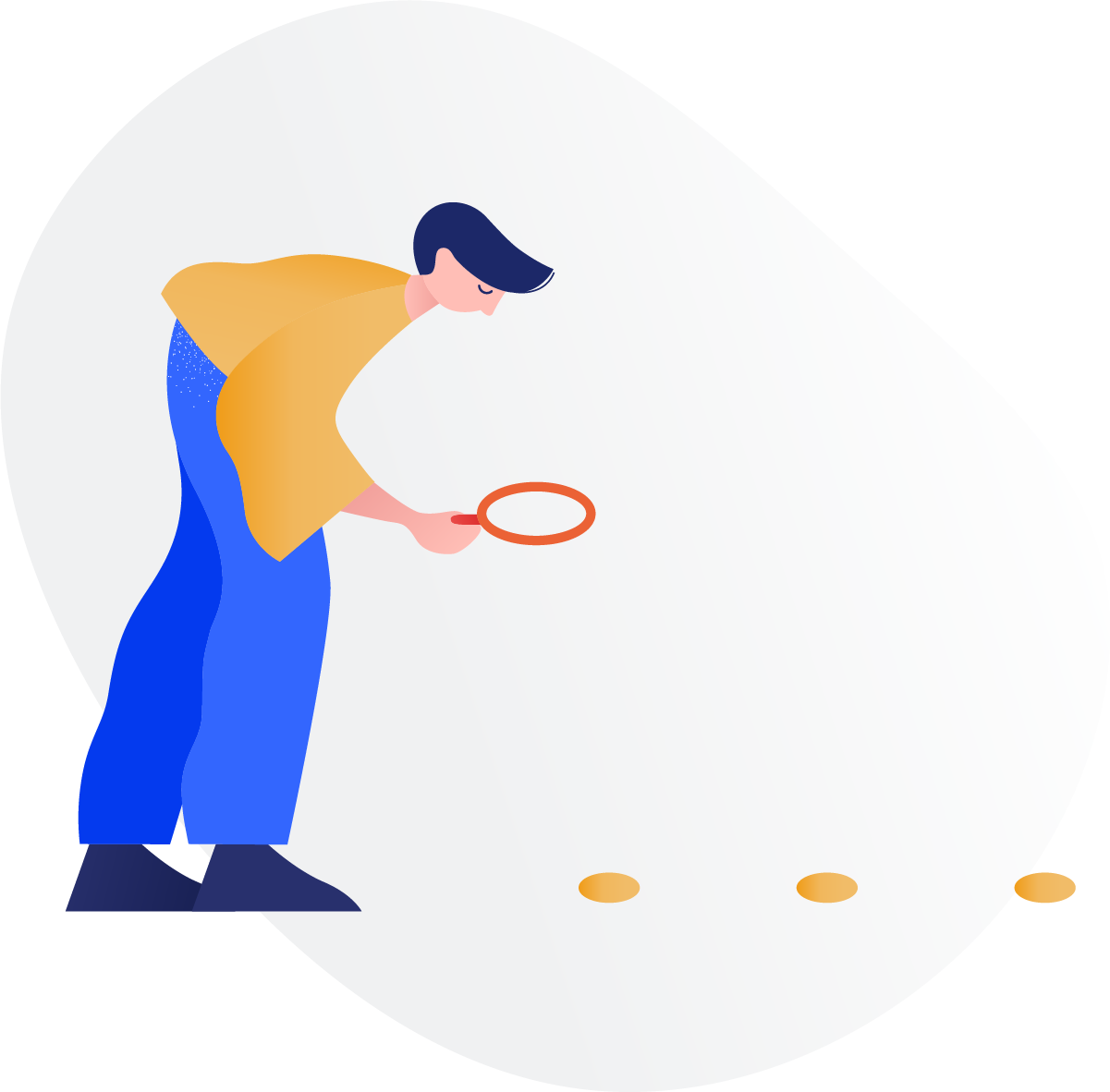
وجه الفتاة! هناك خطأ ما.
أثناء محاولة إضافة العنوان إلى الرف ، حدث خطأ ما :( يرجى إعادة المحاولة لاحقًا!
-
الضبطالضبط
-
مُحَكَّمةمُحَكَّمة
-
نوع العنصرنوع العنصر
-
الموضوعالموضوع
-
السنةمن:-إلى:
-
المزيد من المرشحاتالمزيد من المرشحاتالمصدراللغة
منجز
مرشحات
إعادة تعيين
255,218
نتائج ل
"Accuracy"
صنف حسب:
0449 Comparing Deep Feature Representations to Improve Robustness to Subject Variation in Snore Detection
2019
Introduction Snoring is an indicator of obstructive sleep apnea (OSA), which contributes to cardiovascular disease and mortality. To better study snoring, audio-based snore detection methods using different feature representations have been proposed. However, there is a gap in (1) baseline comparisons of different deep learning features, and (2) analysis of the robustness of snore detection in the presence of subject variation. Through an ablation study, we quantified the effect of features. As a measure of robustness to subject variation, we employed a leave-one-subject-out scheme. Methods We used 1D raw signals or 2D Mel-frequency-cepstrum-coefficients (MFCC) of the signals as inputs to fully connected, convolutional, long-short-term-memory (LSTM) cell-based recurrent, very deep networks (VGG) or combinations of them. The classifiers were support-vector-machines (SVM) or neural networks. The ablation study consists of seven modular combinations of the elements mentioned above. For training, we used 81,207 snore and non-snore 5s- segments from the snore channel of polysomnography (PSG) data obtained from 19 subjects. A leave-one-subject-out scheme, in which each subject is tested using the training data from other subjects, is used to simulate subject variation. We then measure the variation in performance (F1-score) over different subjects using the standard deviation (SD). Results Features learned from 2D convolutional, LSTM, and very deep network (VGG) significantly improve the classification accuracy and robustness of snore detection. Applying these findings, we developed a 2D convolutional LSTM network model that combines spectral and temporal features, resulting in the highest accuracy (mean F1-score = 0.8812) and the second-best robustness. Very deep convolutional networks (VGG-SVM) has the most robust performance (SD of F1-score = 0.0568). Conclusion We provide a baseline comparison to understand the effect of feature representation on snore classification. Besides accuracy, we introduce robustness as another performance metric. Methods with the best accuracy do not necessarily give the best robustness. Features extracted from 2D-convolutional and LSTM network results in the best accuracy, but those from very deep convolutional networks (VGG) have the best robustness. Support (If Any) Supported by Philips Respironics.
Journal Article
High-accuracy wavefront sensing by phase diversity technique with bisymmetric defocuses diversity phase
2017
We investigate a specific diversity phase for phase diversity (PD) phase retrieval, which possesses higher accuracy than common PD, especially for large-scale and high-frequency wavefront sensing. The commonly used PD algorithm employs the image intensities of the focused plane and one defocused plane to build the error metric. Unlike the commonly used PD, we explore a bisymmetric defocuses diversity phase, which employs the image intensities of two symmetrical defocused planes to build the error metric. This kind of diversity phase, named PD-BD (bisymmetric defocuses phase diversity), is analysed with the Cramer-Rao lower bound (CRLB). Statistically, PD-BD shows smaller CRLBs than the commonly used PD, which indicates stronger capacity of phase retrieval. Numerical simulations also verify that PD-BD has higher accuracy of phase retrieval than the commonly used PD when dealing with large-scale and high-frequency wavefront aberrations. To further affirm that PD-BD possesses higher accuracy of wavefront sensing than PD, we also perform a simple verification experiment.
Journal Article
F67 Optimising discriminative ability of performance-based assessments in hd
2018
Background The Clinch Token Transfer Test (C3t) and Step & Stroop Test (SST) are newly developed dual task assessments for Huntington’s Disease (HD). As these are formed of a number of items they produce numerous variables and it is unknown which of these have greatest discriminative ability for each assessment. Aims To use machine learning classifiers to assess the discriminative ability of the tests and to determine which individual aspects are most important to retain. Methods Controls (n=27) and manifest HD (n=36) participants performed the C3t and SST. The C3t records 30 variables including time to complete each item, number of errors made and task costs (the difference in performance between increasingly complex tasks). The SST records 20 variables including number of steps and Stroop accuracy. To determine the discriminatory power of the assessments two classifiers were constructed, one using variables from the C3t and another using variables from the SST. A feature selection algorithm was used to determine which assessment variables were most important to retain. Results The best performing SST classifier had a mean accuracy of 84% using the number of steps in the baseline Step task, the number of correct answers in the Stroop Congruent Baseline and the number of correct answers in the Stroop Incongruent Baseline. The best performing C3t classifier had a mean accuracy of 88% using the time taken for the C3t Dual Task, the time taken for the C3t Triple Task and the task cost (time) between Baseline and Dual Tasks. Conclusions This study suggests that the C3t and SST are reasonably suitable for distinguishing between manifest HD and controls. Furthermore, the assessment complexity can be reduced as the optimal models required a fraction of the scores recorded. Future work will seek to a) reduce the complexity of the tests and b) explore potential test enhancements that may bolster classifier performance.
Journal Article
EP798 Diagnostic accuracy of gynecology imaging reporting and data system in evaluation of adnexal lesions
Introduction/BackgroundConsidering the utility of Gynecologic Imaging Reporting and Data System (GI-RADS)in diagnosing malignant adnexal lesions such as ovarian cancer, we aimed to evaluate the diagnostic performance of this reporting system in differentiating between malignant and benign adnexal lesions.MethodologyIn this cross sectional study, women with suspected adnexal lesions were enrolled. For differentiating of malignant adnexal lesions Grade II & III of GIRADs system were classified as low risk for malignancy and grades IV and V as high risk. Results of histopathologic diagnosis were compared with the results of the mentioned GI-RADS system classification and the diagnosed accuracy of the system was determined. Patients who did not have histopathologic diagnosis were followed up.ResultsIn this study, 197 women with suspected adnexal lesions were evaluated. Frequency of GI-RADS II, III, IV and V were 34.5% (69 cases), 38.0% (76 cases), 19.5% (39 cases) and 6.5% (13 cases) respectively. According to the low and high risk classification of GI-RADS, 72.5% were classified as GIRADs II & III and 26% as GIRADs IV & V respectively. Definitive histopathologic diagnosiswas reported for 158 cases. Histopathologic evaluation indicated that 12 (7.6%)of the masses were malignant and 146 (92.6%) were benign. Comparing with the histopathologicdiagnosis, theGI-RADS system sensitivity, specificity, PPV, NPV, (+) LR and (−) LR were 91.6%, 80.82%, 28.2%, 99.1%, 4.77 and 0.10, respectively. The accuracy of the scoring system was 81.64%.ConclusionGI-RADS system could be useful for clinicians for performing an appropriate clinical management. However, given that the sensitivity of the system is higher than its specificity, it is recommended to design further studies with additional laboratory tests such as CA-125.DisclosureNothing to disclose.
Journal Article
Field-aware User Influence Recommendation Model Based on Trust Relationship
2020
In current determining of user influence based on network structure, the overall importance of user node in network usually attracts great attention. However, the significance of the users in specific field has not been fully studied, which result in low accuracy and reliability in user influence measurement. In order to resolve these problems, this research proposes a field-aware user influence model, which constructs user influence for specific fields, and analyses the global influence of users in the whole network on a network structure basis. The model, assisted by historical behaviour data, not only considers the spread of user influence, but also takes the relationship between user influence and fields into account. Referring to the global influence, the model is able to optimize the composition of user influence, and further improve the accuracy of recommendation. The experiments based on real data sets conducted in this research unexceptionally proved that the field-aware user influence model proposed in this paper, compared with other methods, can effectively improve the recommendation accuracy.
Journal Article
北斗三号全球导航卫星系统全球广播电离层延迟修正模型(BDGIM)应用性能评估
في
Accuracy
2021
2020年6月23日,我国北斗三号全球导航卫星系统正式完成星座全球组网。北斗三号全球导航卫星系统采用新一代全球广播电离层延迟修正模型(BDGIM),为用户提供电离层延迟改正服务。本文利用高精度全球电离层格网(GIM)以及实测BDS/GPS数据提供的电离层TEC作为参考,从延迟改正精度及北斗单频伪距单点定位应用、模型系数性能等方面,对北斗三号系统组网前后(2020年5月1日至2020年7月20日)BDGIM模型的改正精度等应用性能进行了分析与研究,并将其与美国GPS播发的Klobuchar模型和北斗二号卫星导航系统播发的BDS Klobuchar模型进行对比。研究表明,BDGIM模型在对北斗三号系统组网完成前后电离层延迟修正精度没有发生显著变化。上述时段内,以国际GNSS服务(IGS)发布的最终GIM产品为参考,BDGIM模型在中国区域、亚太地区和全球范围内的电离层修正百分比分别达到84.45%、74.74%和64.57%;以选取的全球83个GNSS检测站BDS、GPS双频数据实测电离层TEC为参考,BDGIM在中国区域、亚太地区和全球范围内的电离层修正百分比分别为73.12%、70.18%及68.06%;当BDGIM模型应用于北斗单频伪距单点定位时,在中国区域、亚太地区和全球范围内分别实现了2.22、2.66和2.96 m的三维定位精度。
Journal Article
顾及气候差异的区域加权平均温度模型——以中国陕西为例
في
Accuracy
2021
加权平均温度Tm是全球导航卫星系统(GNSS)反演可降水量的关键参数。本文以中国陕西为例,结合欧洲天气预报中心(ECMWF)的再分析数据与3个探空站数据,基于最小二乘原理建立了一种顾及周期性的Tm区域化回归模型。利用陕西省内3个探空站数据进行验证。结果表明,本文所建立的顾及周期的Tm区域模型比传统Bevis模型精度平均提升率为16.1%。另外,针对不同气候类型地区的差异问题,建立了随纬度变化分段线性形式的顾及气候差异的Tm模型,解决了回归模型在不同气候区的适应性问题。与探空数据比较,顾及气候差异的Tm模型其外符合精度(RMS)范围在1.47~2.06 K之间,与Bevis模型比较,精度平均提高率为44.9%,提升效果显著;利用ECMWF数据选取19个格网点对模型进行精度评估。结果表明:平均RMS为3.26 K,最大RMS为3.67 K;平均STD为2.69 K,最大STD为3.19 K。
Journal Article