Catalogue Search | MBRL
نتائج البحث
MBRLSearchResults
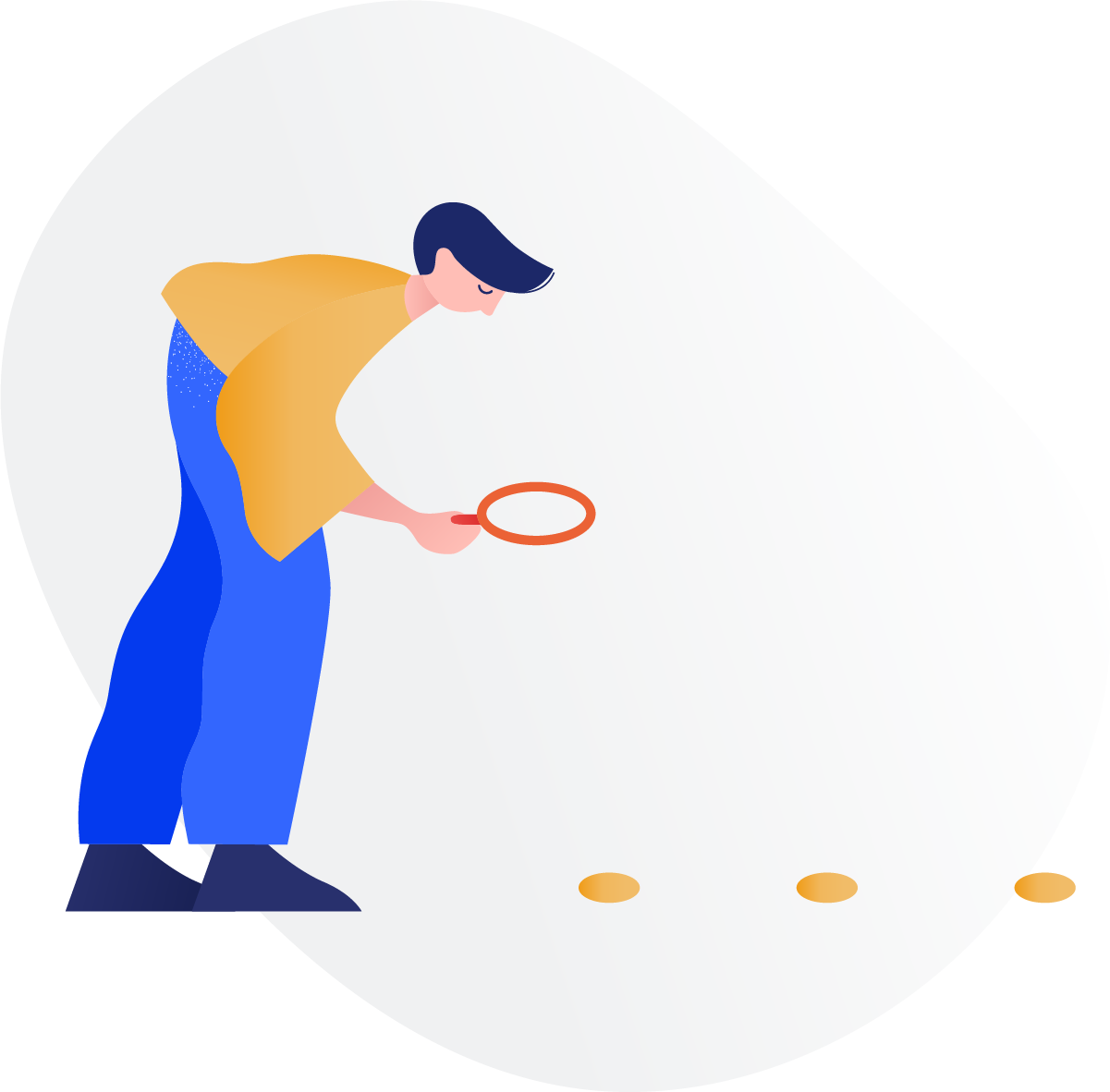
وجه الفتاة! هناك خطأ ما.
أثناء محاولة إضافة العنوان إلى الرف ، حدث خطأ ما :( يرجى إعادة المحاولة لاحقًا!
-
الضبطالضبط
-
مُحَكَّمةمُحَكَّمة
-
نوع العنصرنوع العنصر
-
الموضوعالموضوع
-
السنةمن:-إلى:
-
المزيد من المرشحاتالمزيد من المرشحاتالمصدراللغة
منجز
مرشحات
إعادة تعيين
49,980
نتائج ل
"Heterogeneity"
صنف حسب:
Correction: Evaluation and evolution of bank efficiency considering heterogeneity technology: An empirical study from China
2019
[This corrects the article DOI: 10.1371/journal.pone.0204559.].
Journal Article
More years, more technologies: Aging in the digital era
2018
This special issue presents research on the role of digital health and communication technologies in later life. Drawing from the observation that people’s longer lives are affected by digital technologies to varying extents and recognizing the vast supply of traditional and digital technologies targeted at older users, we maintain that the principle of aged heterogeneity also manifests itself with respect to the adoption and use of digital technologies. Basing on the findings presented in the articles of the issue, we conclude n this introduction to the issue that the concept of aged heterogeneity and the particularities of old age as a stage of life are still insufficiently incorporated into the design of digital technologies and applications.
Journal Article
Corrigendum: Large-scale heterogeneity of Amazonian phenology revealed from 26-year long AVHRR/NDVI time-series
2013
The full text of this article is available in the PDF provided.
Journal Article
Ovarian Cancer: A Heterogeneous Disease
بواسطة
Scoazec, Jean-Yves
,
Kossaï, Myriam
,
Leary, Alexandra
في
Biomarkers, Tumor - genetics
,
Carcinoma - classification
,
Carcinoma - diagnosis
2018
Ovarian cancer encompasses a collection of neoplasms with distinct clinicopathological and molecular features and prognosis. Despite there being a variety of ovarian cancer subtypes, these are treated as a single disease. Tremendous efforts have been made to characterize these subtypes and identify tumoral pathways and potential biomarkers for therapeutic strategies. As in other cancer types, tumor heterogeneity appears to be very high across subtypes and within a single tumor, representing a major cause of treatment failure. We describe the morphological and molecular heterogeneity among ovarian cancers and discuss recent advances in our understanding of intratumor heterogeneity.
Journal Article
Author Correction: Liquid versus tissue biopsy for detecting acquired resistance and tumor heterogeneity in gastrointestinal cancers
2019
An amendment to this paper has been published and can be accessed via a link at the top of the paper.
Journal Article
Correction: Taxonomic and functional heterogeneity of the gill microbiome in a symbiotic coastal mangrove lucinid species
2020
An amendment to this paper has been published and can be accessed via a link at the top of the paper.
Journal Article
Heterogeneity–disease relationship in the human microbiome-associated diseases
2020
ABSTRACT
Space is a critical and also challenging frontier in human microbiome research. It has been found that lack of consideration of scales beyond individual and ignoring of microbe dispersal are two crucial roadblocks in preventing deep understanding of the spatial heterogeneity of human microbiome. Assessing and interpreting the heterogeneity and dispersal in microbiomes explicitly are particularly challenging, but implicit approaches such as Taylor's power law (TPL) can be rather effective. Based on TPL, which achieved a rare status of ecological laws, we introduce a general methodology for characterizing the spatial heterogeneity of microbiome (i.e. characterization of microbial spatial distribution) and further apply it for investigating the heterogeneity–disease relationship (HDR) via analyzing a big dataset of 26 MAD (microbiome-associated disease) studies covering nearly all high-profile MADs including obesity, diabetes and gout. It was found that in majority of the MAD cases, the microbiome was sufficiently resilient to endure the disease disturbances. Specifically, in ∼10–16% cases, disease effects were significant—the healthy and diseased cohorts exhibited statistically significant differences in the TPL heterogeneity parameters. We further compared HDR with classic diversity–disease relationship (DDR) and explained their mechanistic differences. Both HDR and DDR cross-verified remarkable resilience of the human microbiomes against MADs.
Since assessing and interpreting the heterogeneity and dispersal in microbiomes explicitly are particularly challenging, we introduce an implicit approach, i.e. Taylor's power law, to investigate the heterogeneity–disease relationship in the human microbiome-associated diseases.
Journal Article
Ultra‐high sensitivity mass spectrometry quantifies single‐cell proteome changes upon perturbation
بواسطة
Thielert, Marvin
,
Hoerning, Ole B
,
Theis, Fabian J
في
Cell cycle
,
Chromatography
,
drug perturbation
2022
Single‐cell technologies are revolutionizing biology but are today mainly limited to imaging and deep sequencing. However, proteins are the main drivers of cellular function and in‐depth characterization of individual cells by mass spectrometry (MS)‐based proteomics would thus be highly valuable and complementary. Here, we develop a robust workflow combining miniaturized sample preparation, very low flow‐rate chromatography, and a novel trapped ion mobility mass spectrometer, resulting in a more than 10‐fold improved sensitivity. We precisely and robustly quantify proteomes and their changes in single, FACS‐isolated cells. Arresting cells at defined stages of the cell cycle by drug treatment retrieves expected key regulators. Furthermore, it highlights potential novel ones and allows cell phase prediction. Comparing the variability in more than 430 single‐cell proteomes to transcriptome data revealed a stable‐core proteome despite perturbation, while the transcriptome appears stochastic. Our technology can readily be applied to ultra‐high sensitivity analyses of tissue material, posttranslational modifications, and small molecule studies from small cell counts to gain unprecedented insights into cellular heterogeneity in health and disease.
Synopsis
A new ultra‐high sensitivity LC‐MS workflow increases sensitivity by up to two orders of magnitude and enables true single‐cell proteome analysis. In‐depth comparison indicates that the single‐cell transcriptome is stochastic while the single‐cell proteome is complete and stable.
A highly optimized data independent acquisition powered single‐cell proteomics workflow including sub‐µl sample preparation, very low flow chromatography and trapped ion mobility mass spectrometry (diaPASEF) is presented.
Single‐cell proteome analysis is performed by injecting cells one‐by‐one across the cell cycle into the LC‐MS and correctly identifies cell states.
Single‐cell proteome information is highly complementary to single‐cell transcriptome information.
At the single‐cell level the proteome is quantitatively and qualitatively stable, while the transcriptome is stochastic.
A new ultra‐high sensitivity LC‐MS workflow increases sensitivity by up to two orders of magnitude and enables true single‐cell proteome analysis. In‐depth comparison indicates that the single‐cell transcriptome is stochastic while the single‐cell proteome is complete and stable.
Journal Article