Catalogue Search | MBRL
نتائج البحث
MBRLSearchResults
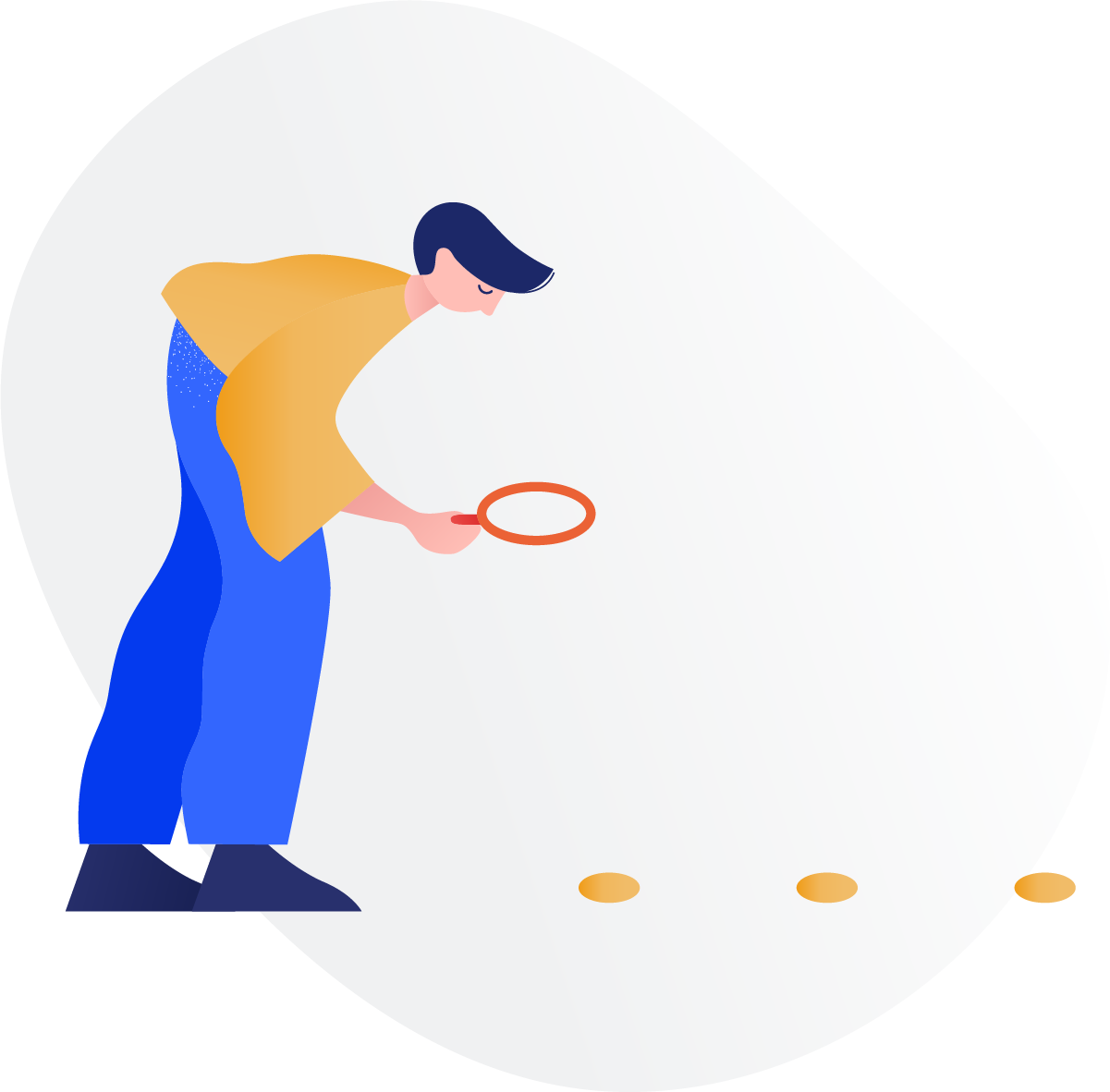
وجه الفتاة! هناك خطأ ما.
أثناء محاولة إضافة العنوان إلى الرف ، حدث خطأ ما :( يرجى إعادة المحاولة لاحقًا!
-
الضبطالضبط
-
مُحَكَّمةمُحَكَّمة
-
مستوى القراءةمستوى القراءة
-
نوع المحتوىنوع المحتوى
-
السنةمن:-إلى:
-
المزيد من المرشحاتالمزيد من المرشحاتنوع العنصرلديه النص الكاملالموضوعالناشرالمصدرالمُهدياللغةمكان النشرالمؤلفينالموقع
منجز
مرشحات
إعادة تعيين
77,699
نتائج ل
"Meta-analysis"
صنف حسب:
The Mass Production of Redundant, Misleading, and Conflicted Systematic Reviews and Meta-analyses
2016
Context: Currently, most systematic reviews and meta-analyses are done retrospectively with fragmented published information. This article aims to explore the growth of published systematic reviews and meta-analyses and to estimate how often they are redundant, misleading, or serving conflicted interests. Methods: Data included information from PubMed surveys and from empirical evaluations of meta-analyses. Findings: Publication of systematic reviews and meta-analyses has increased rapidly. In the period January 1, 1986, to December 4, 2015, PubMed tags 266,782 items as \"systematic reviews\" and 58,611 as \"meta-analyses.\" Annual publications between 1991 and 2014 increased 2,728% for systematic reviews and 2,635% for meta-analyses versus only 153% for all PubMed-indexed items. Currently, probably more systematic reviews of trials than new randomized trials are published annually. Most topics addressed by meta-analyses of randomized trials have overlapping, redundant meta-analyses; same-topic meta-analyses may exceed 20 sometimes. Some fields produce massive numbers of meta-analyses; for example, 185 meta-analyses of antidepressants for depression were published between 2007 and 2014. These meta-analyses are often produced either by industry employees or by authors with industry ties and results are aligned with sponsor interests. China has rapidly become the most prolific producer of English-language, PubMed-indexed meta-analyses. The most massive presence of Chinese meta-analyses is on genetic associations (63% of global production in 2014), where almost all results are misleading since they combine fragmented information from mostly abandoned era of candidate genes. Furthermore, many contracting companies working on evidence synthesis receive industry contracts to produce meta-analyses, many of which probably remain unpublished. Many other meta-analyses have serious flaws. Of the remaining, most have weak or insufficient evidence to inform decision making. Few systematic reviews and meta-analyses are both non-misleading and useful. Conclusions: The production of systematic reviews and meta-analyses has reached epidemic proportions. Possibly, the large majority of produced systematic reviews and meta-analyses are unnecessary, misleading, and/or conflicted.
Journal Article
A Guide to Conducting a Meta-Analysis with Non-Independent Effect Sizes
2019
Conventional meta-analytic procedures assume that effect sizes are independent. When effect sizes are not independent, conclusions based on these conventional procedures can be misleading or even wrong. Traditional approaches, such as averaging the effect sizes and selecting one effect size per study, are usually used to avoid the dependence of the effect sizes. These ad-hoc approaches, however, may lead to missed opportunities to utilize all available data to address the relevant research questions. Both multivariate meta-analysis and three-level meta-analysis have been proposed to handle non-independent effect sizes. This paper gives a brief introduction to these new techniques for applied researchers. The first objective is to highlight the benefits of using these methods to address non-independent effect sizes. The second objective is to illustrate how to apply these techniques with real data in R and Mplus. Researchers may modify the sample R and Mplus code to fit their data.
Journal Article
What One Hundred Years of Research Says About the Effects of Ability Grouping and Acceleration on K-12 Students' Academic Achievement: Findings of Two Second-Order Meta-Analyses
بواسطة
Olszewski-Kubilius, Paula
,
Makel, Matthew C.
,
Steenbergen-Hu, Saiying
في
Ability Grouping
,
Academic Ability
,
Academic Achievement
2016
Two second-order meta-analyses synthesized approximately 100 years of research on the effects of ability grouping and acceleration on K-12 students' academic achievement. Outcomes of 13 ability grouping meta-analyses showed that students benefitedfrom within-class grouping (0.19≤g≤0.30), cross-grade subject grouping (g = 0.26), and special grouping for the gifted (g = 0.3 7), but did not benefitfrom between-class grouping (0.04≤g≤0.06); the effects did not vary for high-, medium-, and low-ability students. Three acceleration meta-analyses showed that accelerated students significantly outperformed their nonaccelerated same-age peers (g = 0.70) but did not differ significantly from nonaccelerated older peers (g = 0.09). Three other meta-analyses that aggregated outcomes across specific forms of acceleration found that acceleration appeared to have a positive, moderate, and statistically significant impact on students' academic achievement (g = 0.42).
Journal Article
Methodological issues and advances in biological meta-analysis
بواسطة
Nakagawa, Shinichi
,
Santos, Eduardo S. A.
في
Analysis
,
Animal Ecology
,
Biomedical and Life Sciences
2012
Meta-analysis has changed the way researchers conduct literature reviews not only in medical and social sciences but also in biological sciences. Meta-analysis in biological sciences, especially in ecology and evolution (which we refer to as ‘biological’ meta-analysis) faces somewhat different methodological problems from its counterparts in medical and social sciences, where meta-analytic techniques were originally developed. The main reason for such differences is that biological meta-analysis often integrates complex data composed of multiple strata with, for example, different measurements and a variety of species. Here, we review methodological issues and advancements in biological meta-analysis, focusing on three topics: (1) non-independence arising from multiple effect sizes obtained in single studies and from phylogenetic relatedness, (2) detecting and accounting for heterogeneity, and (3) identifying publication bias and measuring its impact. We show how the marriage between mixed-effects (hierarchical/multilevel) models and phylogenetic comparative methods has resolved most of the issues under discussion. Furthermore, we introduce the concept of across-study and within-study meta-analysis, and propose how the use of within-study meta-analysis can improve many empirical studies typical of ecology and evolution.
Journal Article
Graphical methods and numerical summaries for presenting results from multiple-treatment meta-analysis: an overview and tutorial
بواسطة
Ades, A.E
,
Ioannidis, John P.A
,
Salanti, Georgia
في
Bayes Theorem
,
Bayesian meta-analysis
,
Biological and medical sciences
2011
Abstract Objective To present some simple graphical and quantitative ways to assist interpretation and improve presentation of results from multiple-treatment meta-analysis (MTM). Study Design and Setting We reanalyze a published network of trials comparing various antiplatelet interventions regarding the incidence of serious vascular events using Bayesian approaches for random effects MTM, and we explore the advantages and drawbacks of various traditional and new forms of quantitative displays and graphical presentations of results. Results We present the results under various forms, conventionally based on the mean of the distribution of the effect sizes; based on predictions; based on ranking probabilities; and finally, based on probabilities to be within an acceptable range from a reference. We show how to obtain and present results on ranking of all treatments and how to appraise the overall ranks. Conclusions Bayesian methodology offers a multitude of ways to present results from MTM models, as it enables a natural and easy estimation of all measures based on probabilities, ranks, or predictions.
Journal Article
Heterogeneity in ecological and evolutionary meta-analyses: its magnitude and implications
بواسطة
Grueber, Catherine E.
,
Lagisz, Malgorzata
,
Santos, Eduardo S. A.
في
Benchmarks
,
Bias
,
Biological Evolution
2016
Meta-analysis is the gold standard for synthesis in ecology and evolution. Together with estimating overall effect magnitudes, meta-analyses estimate differences between effect sizes via heterogeneity statistics. It is widely hypothesized that heterogeneity will be present in ecological/evolutionary meta-analyses due to the system-specific nature of biological phenomena. Despite driving recommended best practices, the generality of heterogeneity in ecological data has never been systematically reviewed. We reviewed 700 studies, finding 325 that used formal meta-analysis, of which total heterogeneity was reported in fewer than 40%. We used second-order meta-analysis to collate heterogeneity statistics from 86 studies. Our analysis revealed that the median and mean heterogeneity, expressed as I², are 84.67% and 91.69%, respectively. These estimates are well above \"high\" heterogeneity (i.e., 75%), based on widely adopted benchmarks. We encourage reporting heterogeneity in the forms of I² and the estimated variance components (e.g., τ²) as standard practice. These statistics provide vital insights in to the degree to which effect sizes vary, and provide the statistical support for the exploration of predictors of effect-size magnitude. Along with standard meta-regression techniques that fit moderator variables, multi-level models now allow partitioning of heterogeneity among correlated (e.g., phylogenetic) structures that exist within data.
Journal Article
Active learning increases student performance in science, engineering, and mathematics
بواسطة
Eddy, Sarah L.
,
Jordt, Hannah
,
Smith, Michelle K.
في
Achievement
,
Active learning
,
Biology
2014
To test the hypothesis that lecturing maximizes learning and course performance, we metaanalyzed 225 studies that reported data on examination scores or failure rates when comparing student performance in undergraduate science, technology, engineering, and mathematics (STEM) courses under traditional lecturing versus active learning. The effect sizes indicate that on average, student performance on examinations and concept inventories increased by 0.47 SDs under active learning (n = 158 studies), and that the odds ratio for failing was 1.95 under traditional lecturing (n = 67 studies). These results indicate that average examination scores improved by about 6% in active learning sections, and that students in classes with traditional lecturing were 1.5 times more likely to fail than were students in classes with active learning. Heterogeneity analyses indicated that both results hold across the STEM disciplines, that active learning increases scores on concept inventories more than on course examinations, and that active learning appears effective across all class sizes—although the greatest effects are in small (n ≤ 50) classes. Trim and fill analyses and fail-safe n calculations suggest that the results are not due to publication bias. The results also appear robust to variation in the methodological rigor of the included studies, based on the quality of controls over student quality and instructor identity. This is the largest and most comprehensive metaanalysis of undergraduate STEM education published to date. The results raise questions about the continued use of traditional lecturing as a control in research studies, and support active learning as the preferred, empirically validated teaching practice in regular classrooms.
Journal Article
Mega‐analysis methods in ENIGMA: The experience of the generalized anxiety disorder working group
بواسطة
Perino, Michael T.
,
Lueken, Ulrike
,
Balderston, Nicholas L.
في
Anxiety
,
Anxiety disorders
,
Anxiety Disorders - diagnostic imaging
2022
The ENIGMA group on Generalized Anxiety Disorder (ENIGMA‐Anxiety/GAD) is part of a broader effort to investigate anxiety disorders using imaging and genetic data across multiple sites worldwide. The group is actively conducting a mega‐analysis of a large number of brain structural scans. In this process, the group was confronted with many methodological challenges related to study planning and implementation, between‐country transfer of subject‐level data, quality control of a considerable amount of imaging data, and choices related to statistical methods and efficient use of resources. This report summarizes the background information and rationale for the various methodological decisions, as well as the approach taken to implement them. The goal is to document the approach and help guide other research groups working with large brain imaging data sets as they develop their own analytic pipelines for mega‐analyses.
This report summarizes the background information and rationale for the various methodological decisions made by the ENIGMA—GAD group. The aim of this work is to help guide other research groups working with large brain imaging data sets.
Journal Article