تفاصيل الأصول
MbrlCatalogueTitleDetail
هل ترغب في حجز الكتاب؟
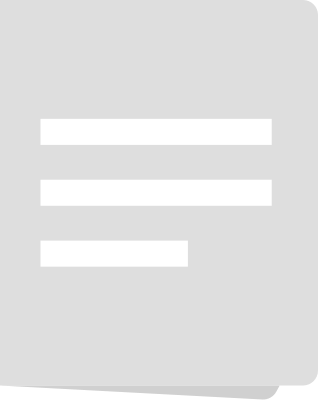
TEsoNet: knowledge transfer in surgical phase recognition from laparoscopic sleeve gastrectomy to the laparoscopic part of Ivor–Lewis esophagectomy
بواسطة
Babic, B.
, Rus, D.
, Fuchs, H. F.
, Witkowski, E.
, Eckhoff, J. A.
, Müller, D. T.
, Meireles, O.
, Rosman, G.
, Hashimoto, D. A.
, Bruns, C.
, Ban, Y.
في
2022 EAES Oral
/ Abdominal Surgery
/ Algorithms
/ Alliances
/ Annotations
/ Artificial Intelligence
/ Authorship
/ Datasets
/ Efficiency
/ Endoscopy
/ Esophageal Neoplasms - surgery
/ Esophagectomy - methods
/ Gastrectomy
/ Gastroenterology
/ Gastrointestinal surgery
/ Gynecology
/ Hepatology
/ Humans
/ Knowledge
/ Laboratories
/ Laparoscopy
/ Laparoscopy - methods
/ Medicine
/ Medicine & Public Health
/ Patient safety
/ Proctology
/ Retrospective Studies
/ Surgery
2023
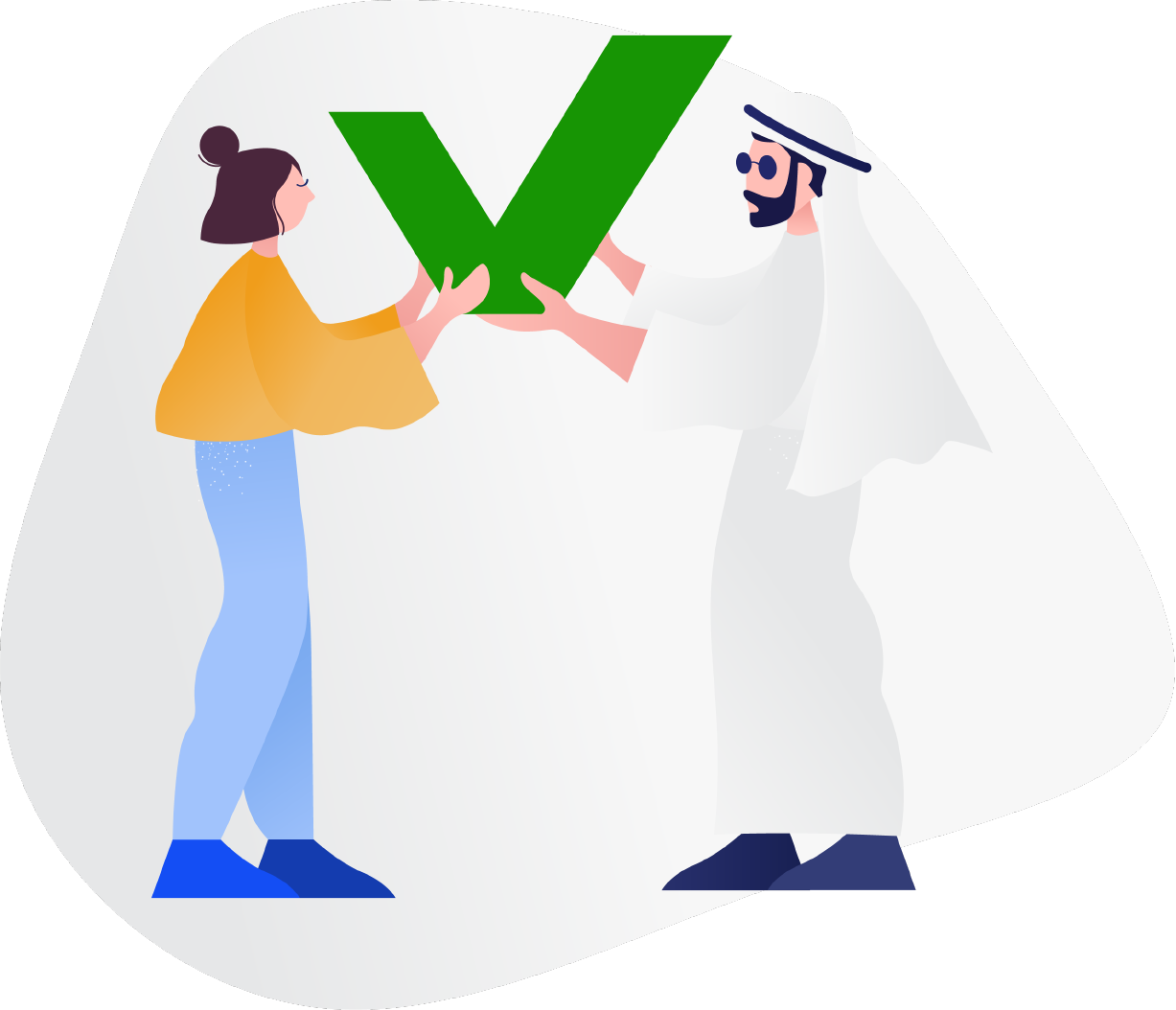
لقد وضعنا الحجز لك!
بالمناسبة ، لماذا لا تستكشف الفعاليات التي يمكنك حضورها عند زيارتك للمكتبة لإستلام كتبك
أنت حاليًا في قائمة الانتظار لالتقاط هذا الكتاب. سيتم إخطارك بمجرد انتهاء دورك في التقاط الكتاب
هل أنت متأكد أنك تريد إزالة الكتاب من الرف؟
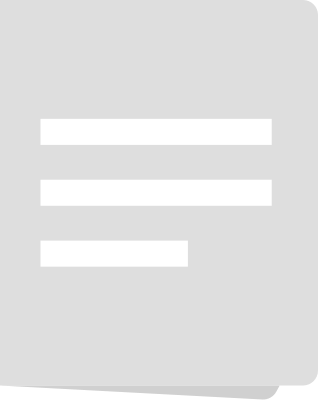
TEsoNet: knowledge transfer in surgical phase recognition from laparoscopic sleeve gastrectomy to the laparoscopic part of Ivor–Lewis esophagectomy
بواسطة
Babic, B.
, Rus, D.
, Fuchs, H. F.
, Witkowski, E.
, Eckhoff, J. A.
, Müller, D. T.
, Meireles, O.
, Rosman, G.
, Hashimoto, D. A.
, Bruns, C.
, Ban, Y.
في
2022 EAES Oral
/ Abdominal Surgery
/ Algorithms
/ Alliances
/ Annotations
/ Artificial Intelligence
/ Authorship
/ Datasets
/ Efficiency
/ Endoscopy
/ Esophageal Neoplasms - surgery
/ Esophagectomy - methods
/ Gastrectomy
/ Gastroenterology
/ Gastrointestinal surgery
/ Gynecology
/ Hepatology
/ Humans
/ Knowledge
/ Laboratories
/ Laparoscopy
/ Laparoscopy - methods
/ Medicine
/ Medicine & Public Health
/ Patient safety
/ Proctology
/ Retrospective Studies
/ Surgery
2023
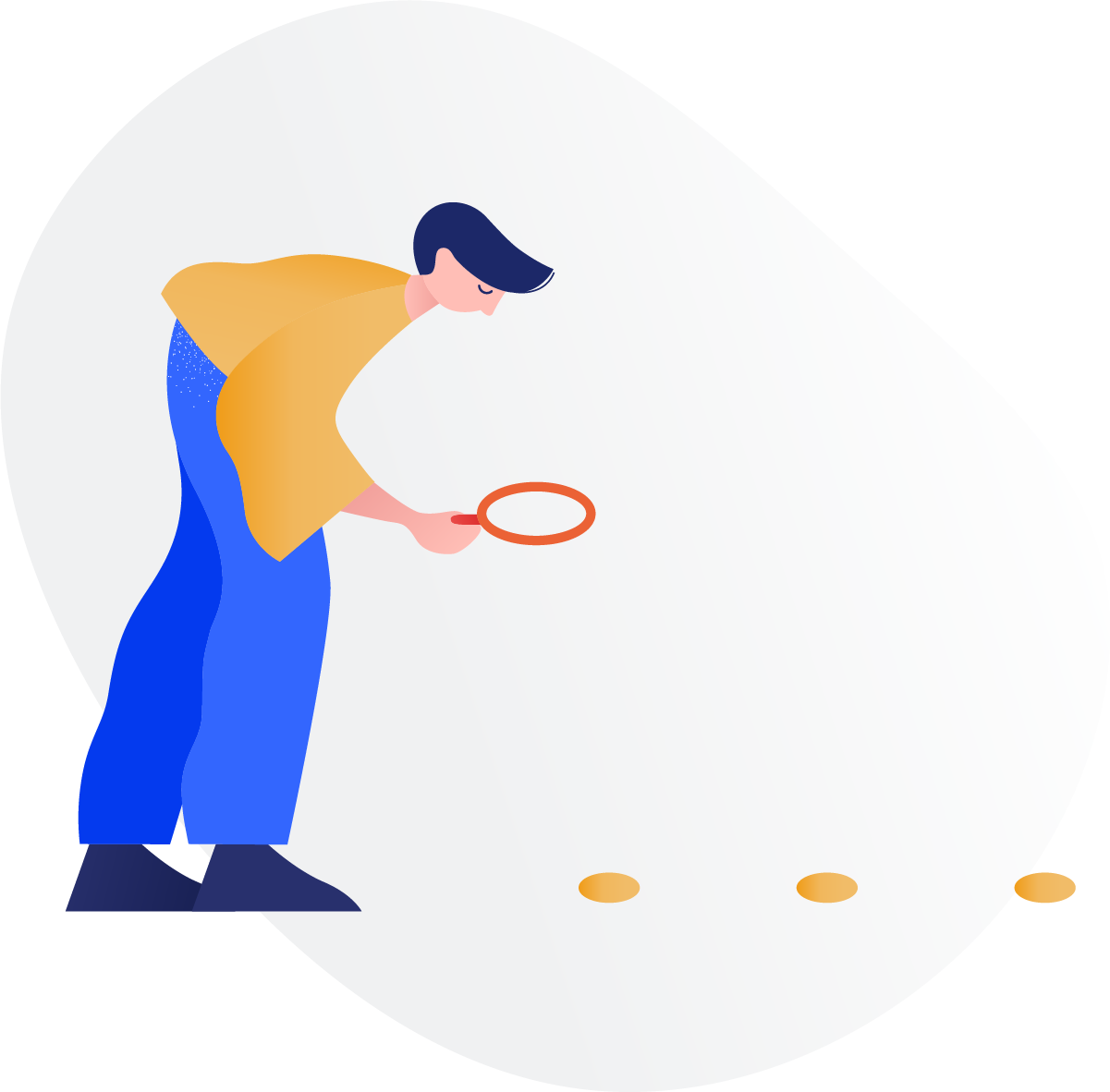
وجه الفتاة! هناك خطأ ما.
أثناء محاولة إزالة العنوان من الرف ، حدث خطأ ما :( يرجى إعادة المحاولة لاحقًا!
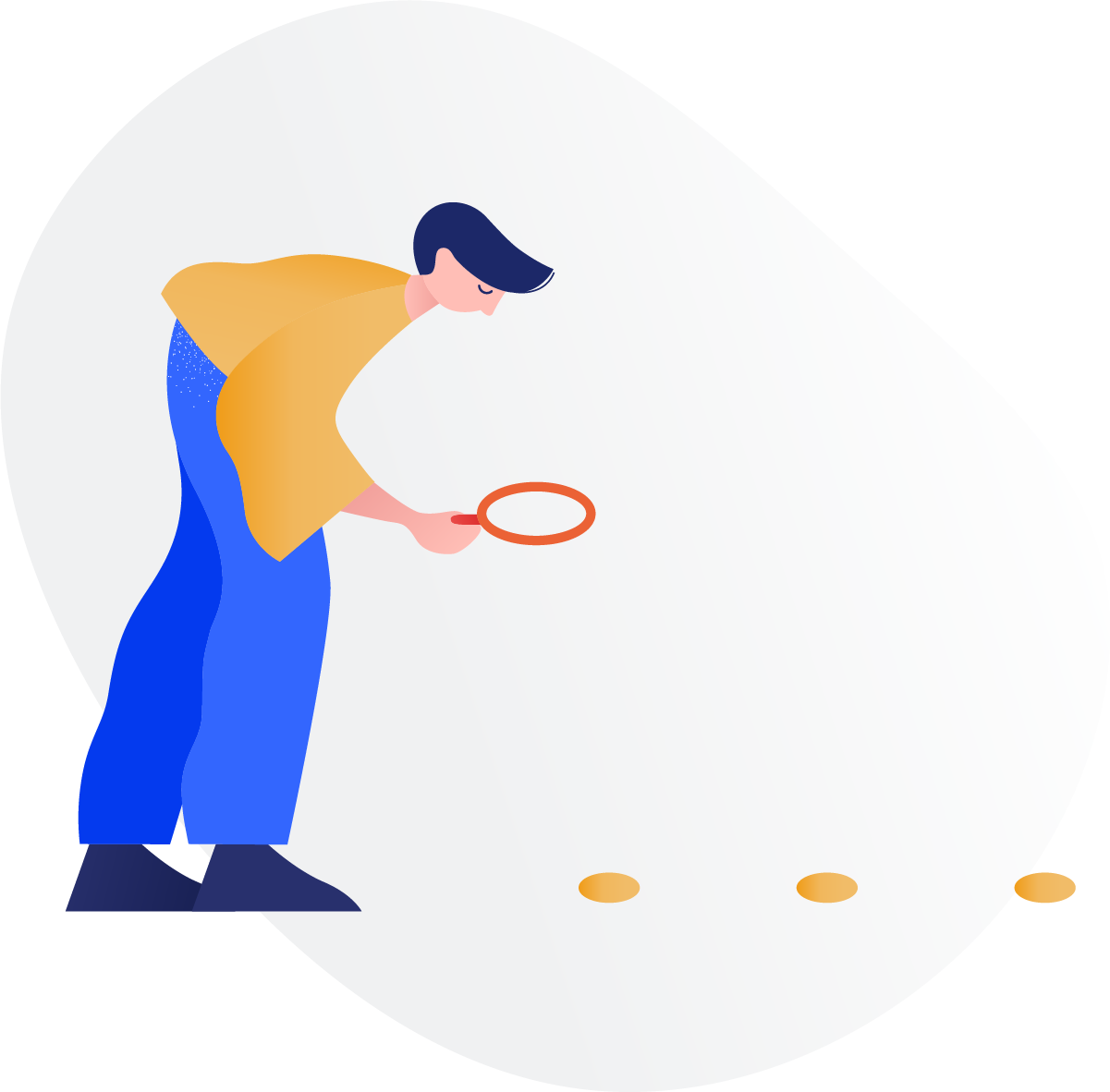
وجه الفتاة! هناك خطأ ما.
أثناء محاولة إضافة العنوان إلى الرف ، حدث خطأ ما :( يرجى إعادة المحاولة لاحقًا!
هل تريد طلب الكتاب؟
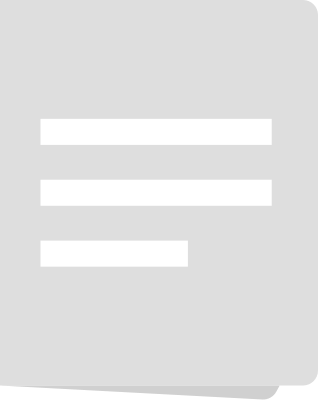
TEsoNet: knowledge transfer in surgical phase recognition from laparoscopic sleeve gastrectomy to the laparoscopic part of Ivor–Lewis esophagectomy
بواسطة
Babic, B.
, Rus, D.
, Fuchs, H. F.
, Witkowski, E.
, Eckhoff, J. A.
, Müller, D. T.
, Meireles, O.
, Rosman, G.
, Hashimoto, D. A.
, Bruns, C.
, Ban, Y.
في
2022 EAES Oral
/ Abdominal Surgery
/ Algorithms
/ Alliances
/ Annotations
/ Artificial Intelligence
/ Authorship
/ Datasets
/ Efficiency
/ Endoscopy
/ Esophageal Neoplasms - surgery
/ Esophagectomy - methods
/ Gastrectomy
/ Gastroenterology
/ Gastrointestinal surgery
/ Gynecology
/ Hepatology
/ Humans
/ Knowledge
/ Laboratories
/ Laparoscopy
/ Laparoscopy - methods
/ Medicine
/ Medicine & Public Health
/ Patient safety
/ Proctology
/ Retrospective Studies
/ Surgery
2023
يرجى العلم أن الكتاب الذي طلبته لا يمكن استعارته. إذا كنت ترغب في إستعارة هذا الكتاب ، يمكنك حجز نسخة أخرى
TEsoNet: knowledge transfer in surgical phase recognition from laparoscopic sleeve gastrectomy to the laparoscopic part of Ivor–Lewis esophagectomy

Journal Article
TEsoNet: knowledge transfer in surgical phase recognition from laparoscopic sleeve gastrectomy to the laparoscopic part of Ivor–Lewis esophagectomy
2023
اطلب الآن
واختر طريقة الاستلام
نظرة عامة
Background
Surgical phase recognition using computer vision presents an essential requirement for artificial intelligence-assisted analysis of surgical workflow. Its performance is heavily dependent on large amounts of annotated video data, which remain a limited resource, especially concerning highly specialized procedures. Knowledge transfer from common to more complex procedures can promote data efficiency. Phase recognition models trained on large, readily available datasets may be extrapolated and transferred to smaller datasets of different procedures to improve generalizability. The conditions under which transfer learning is appropriate and feasible remain to be established.
Methods
We defined ten operative phases for the laparoscopic part of Ivor-Lewis Esophagectomy through expert consensus. A dataset of 40 videos was annotated accordingly. The knowledge transfer capability of an established model architecture for phase recognition (CNN + LSTM) was adapted to generate a “Transferal Esophagectomy Network” (TEsoNet) for co-training and transfer learning from laparoscopic Sleeve Gastrectomy to the laparoscopic part of Ivor-Lewis Esophagectomy, exploring different training set compositions and training weights.
Results
The explored model architecture is capable of accurate phase detection in complex procedures, such as Esophagectomy, even with low quantities of training data. Knowledge transfer between two upper gastrointestinal procedures is feasible and achieves reasonable accuracy with respect to operative phases with high procedural overlap.
Conclusion
Robust phase recognition models can achieve reasonable yet phase-specific accuracy through transfer learning and co-training between two related procedures, even when exposed to small amounts of training data of the target procedure. Further exploration is required to determine appropriate data amounts, key characteristics of the training procedure and temporal annotation methods required for successful transferal phase recognition. Transfer learning across different procedures addressing small datasets may increase data efficiency. Finally, to enable the surgical application of AI for intraoperative risk mitigation, coverage of rare, specialized procedures needs to be explored.
Graphical abstract
الناشر
Springer US,Springer Nature B.V
موضوع
يستخدم هذا الموقع ملفات تعريف الارتباط لضمان حصولك على أفضل تجربة على موقعنا.