تفاصيل الأصول
MbrlCatalogueTitleDetail
هل ترغب في حجز الكتاب؟
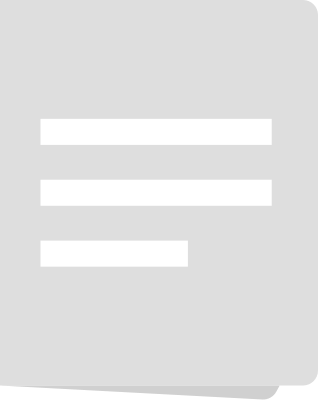
Deep neural networks to recover unknown physical parameters from oscillating time series
بواسطة
Budker, Dmitry
, Garcon, Antoine
, Vexler, Julian
, Kramer, Stefan
في
Analysis
/ Artificial neural networks
/ Biology and Life Sciences
/ Computer and Information Sciences
/ Curve fitting
/ Data processing
/ Discovery and exploration
/ Engineering and Technology
/ Information processing
/ Knowledge
/ Least squares
/ Neural networks
/ NMR
/ Noise reduction
/ Nuclear magnetic resonance
/ Oscillation
/ Outer space
/ Parameters
/ Pattern recognition
/ Physical properties
/ Physical Sciences
/ Physicists
/ Physics
/ Regression
/ Research and Analysis Methods
/ Signal processing
/ Time series
/ Time-series analysis
2022
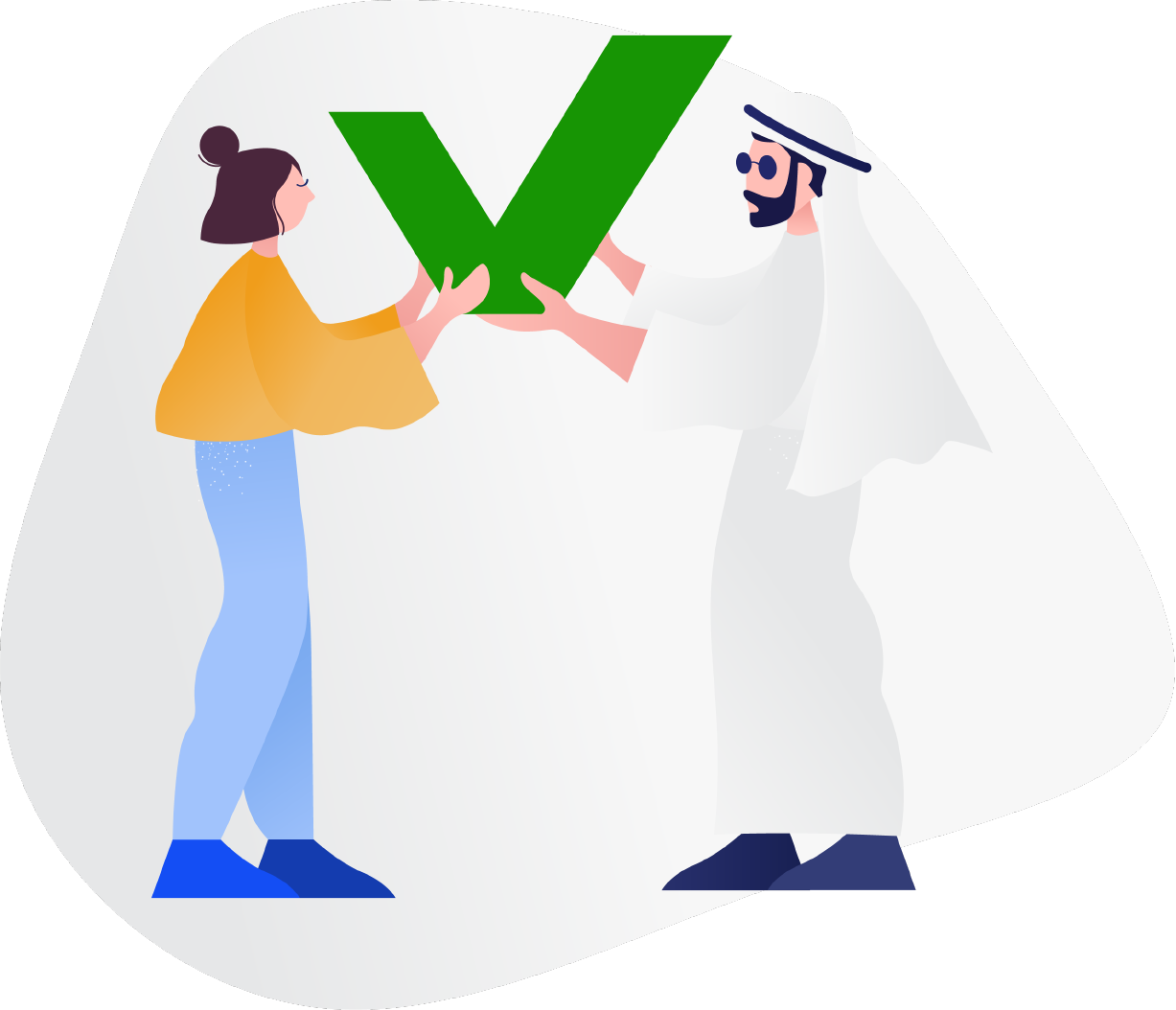
لقد وضعنا الحجز لك!
بالمناسبة ، لماذا لا تستكشف الفعاليات التي يمكنك حضورها عند زيارتك للمكتبة لإستلام كتبك
أنت حاليًا في قائمة الانتظار لالتقاط هذا الكتاب. سيتم إخطارك بمجرد انتهاء دورك في التقاط الكتاب
هل أنت متأكد أنك تريد إزالة الكتاب من الرف؟
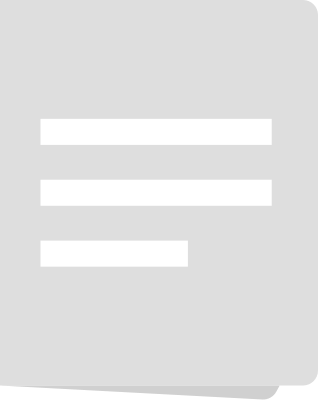
Deep neural networks to recover unknown physical parameters from oscillating time series
بواسطة
Budker, Dmitry
, Garcon, Antoine
, Vexler, Julian
, Kramer, Stefan
في
Analysis
/ Artificial neural networks
/ Biology and Life Sciences
/ Computer and Information Sciences
/ Curve fitting
/ Data processing
/ Discovery and exploration
/ Engineering and Technology
/ Information processing
/ Knowledge
/ Least squares
/ Neural networks
/ NMR
/ Noise reduction
/ Nuclear magnetic resonance
/ Oscillation
/ Outer space
/ Parameters
/ Pattern recognition
/ Physical properties
/ Physical Sciences
/ Physicists
/ Physics
/ Regression
/ Research and Analysis Methods
/ Signal processing
/ Time series
/ Time-series analysis
2022
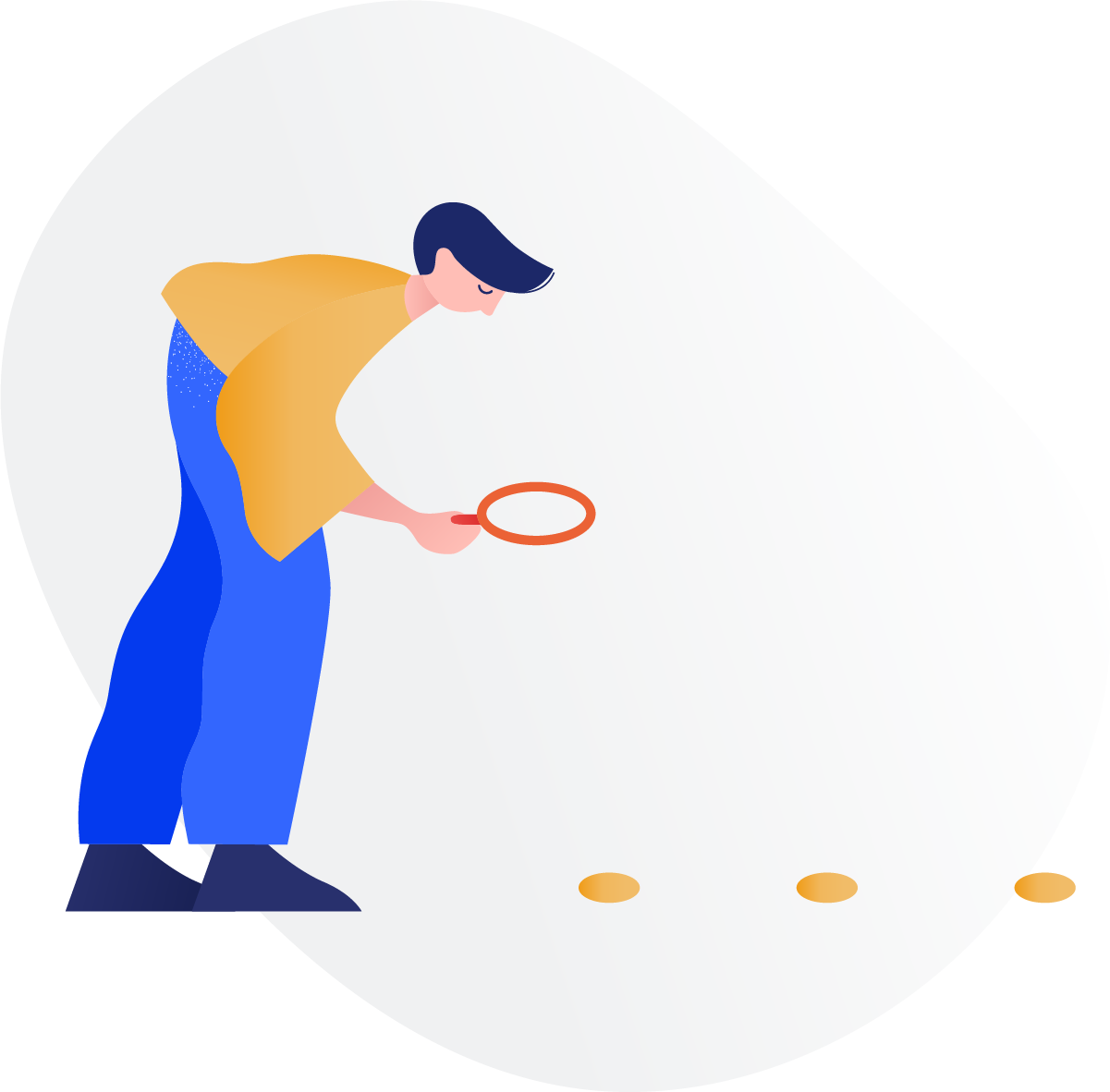
وجه الفتاة! هناك خطأ ما.
أثناء محاولة إزالة العنوان من الرف ، حدث خطأ ما :( يرجى إعادة المحاولة لاحقًا!
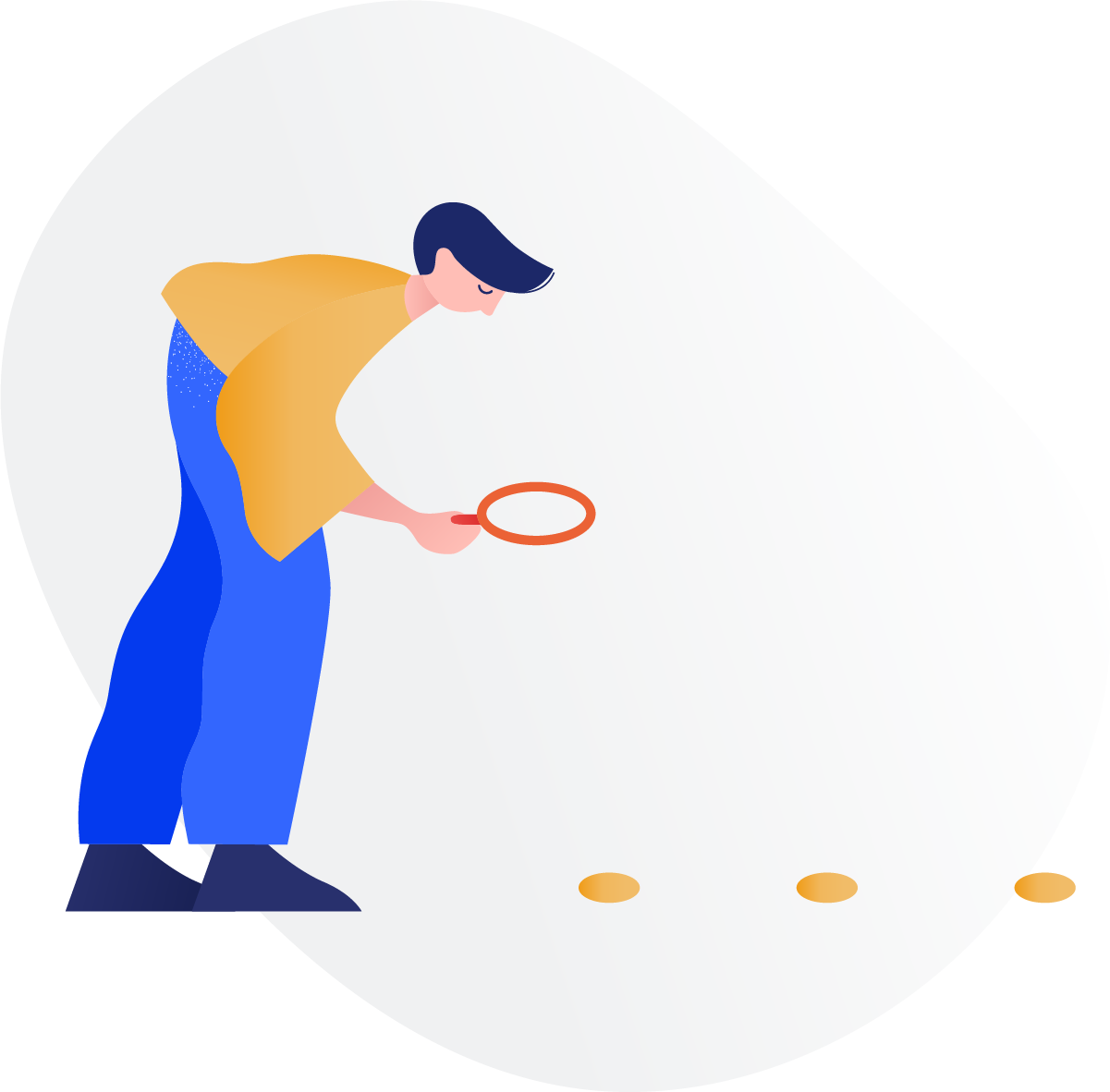
وجه الفتاة! هناك خطأ ما.
أثناء محاولة إضافة العنوان إلى الرف ، حدث خطأ ما :( يرجى إعادة المحاولة لاحقًا!
هل تريد طلب الكتاب؟
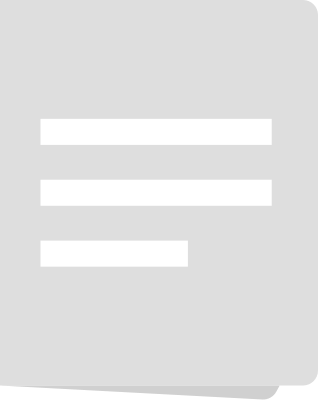
Deep neural networks to recover unknown physical parameters from oscillating time series
بواسطة
Budker, Dmitry
, Garcon, Antoine
, Vexler, Julian
, Kramer, Stefan
في
Analysis
/ Artificial neural networks
/ Biology and Life Sciences
/ Computer and Information Sciences
/ Curve fitting
/ Data processing
/ Discovery and exploration
/ Engineering and Technology
/ Information processing
/ Knowledge
/ Least squares
/ Neural networks
/ NMR
/ Noise reduction
/ Nuclear magnetic resonance
/ Oscillation
/ Outer space
/ Parameters
/ Pattern recognition
/ Physical properties
/ Physical Sciences
/ Physicists
/ Physics
/ Regression
/ Research and Analysis Methods
/ Signal processing
/ Time series
/ Time-series analysis
2022
يرجى العلم أن الكتاب الذي طلبته لا يمكن استعارته. إذا كنت ترغب في إستعارة هذا الكتاب ، يمكنك حجز نسخة أخرى
Deep neural networks to recover unknown physical parameters from oscillating time series

Journal Article
Deep neural networks to recover unknown physical parameters from oscillating time series
2022
اطلب الآن
واختر طريقة الاستلام
نظرة عامة
Deep neural networks are widely used in pattern-recognition tasks for which a human-comprehensible, quantitative description of the data-generating process, cannot be obtained. While doing so, neural networks often produce an abstract (entangled and non-interpretable) representation of the data-generating process. This may be one of the reasons why neural networks are not yet used extensively in physics-experiment signal processing: physicists generally require their analyses to yield quantitative information about the system they study. In this article we use a deep neural network to disentangle components of oscillating time series. To this aim, we design and train the neural network on synthetic oscillating time series to perform two tasks: a regression of the signal latent parameters and signal denoising by an Autoencoder-like architecture. We show that the regression and denoising performance is similar to those of least-square curve fittings with true latent-parameters initial guesses, in spite of the neural network needing no initial guesses at all. We then explore various applications in which we believe our architecture could prove useful for time-series processing, when prior knowledge is incomplete. As an example, we employ the neural network as a preprocessing tool to inform the least-square fits when initial guesses are unknown. Moreover, we show that the regression can be performed on some latent parameters, while ignoring the existence of others. Because the Autoencoder needs no prior information about the physical model, the remaining unknown latent parameters can still be captured, thus making use of partial prior knowledge, while leaving space for data exploration and discoveries.
الناشر
Public Library of Science,Public Library of Science (PLoS)
يستخدم هذا الموقع ملفات تعريف الارتباط لضمان حصولك على أفضل تجربة على موقعنا.