Asset Details
MbrlCatalogueTitleDetail
Do you wish to reserve the book?
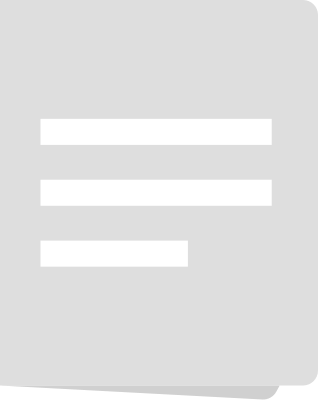
Approximate residual balancing
by
Wager, Stefan
, Athey, Susan
, Imbens, Guido W.
in
Adjustment
/ Assumptions
/ Averages
/ Causal inference
/ Inference
/ Linear models
/ Linearity
/ Parameters
/ Potential outcomes
/ Pretreatment
/ Propensity
/ Propensity score
/ Random variables
/ Regression analysis
/ Regression models
/ Sparse estimation
2018
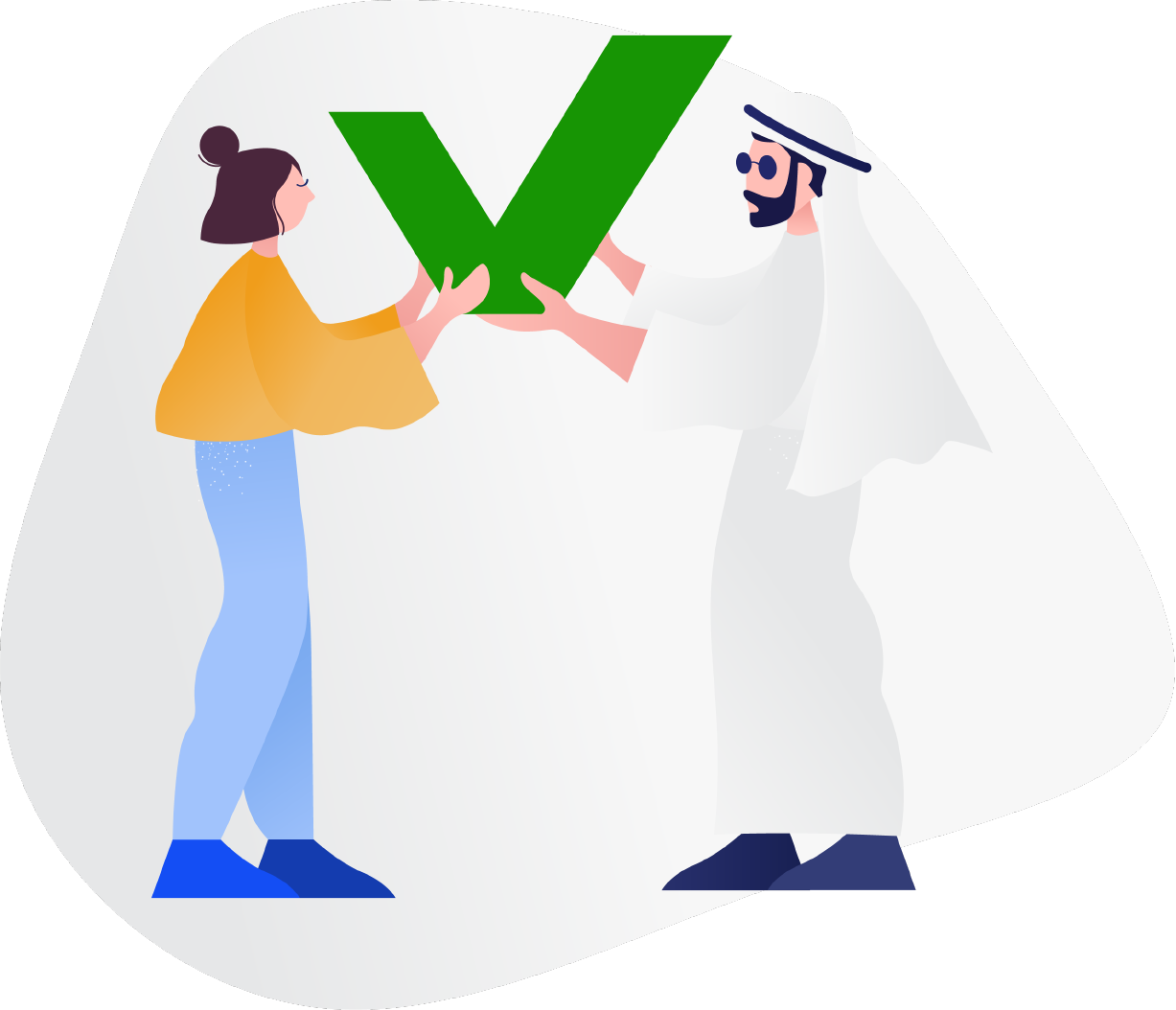
Hey, we have placed the reservation for you!
By the way, why not check out events that you can attend while you pick your title.
You are currently in the queue to collect this book. You will be notified once it is your turn to collect the book.
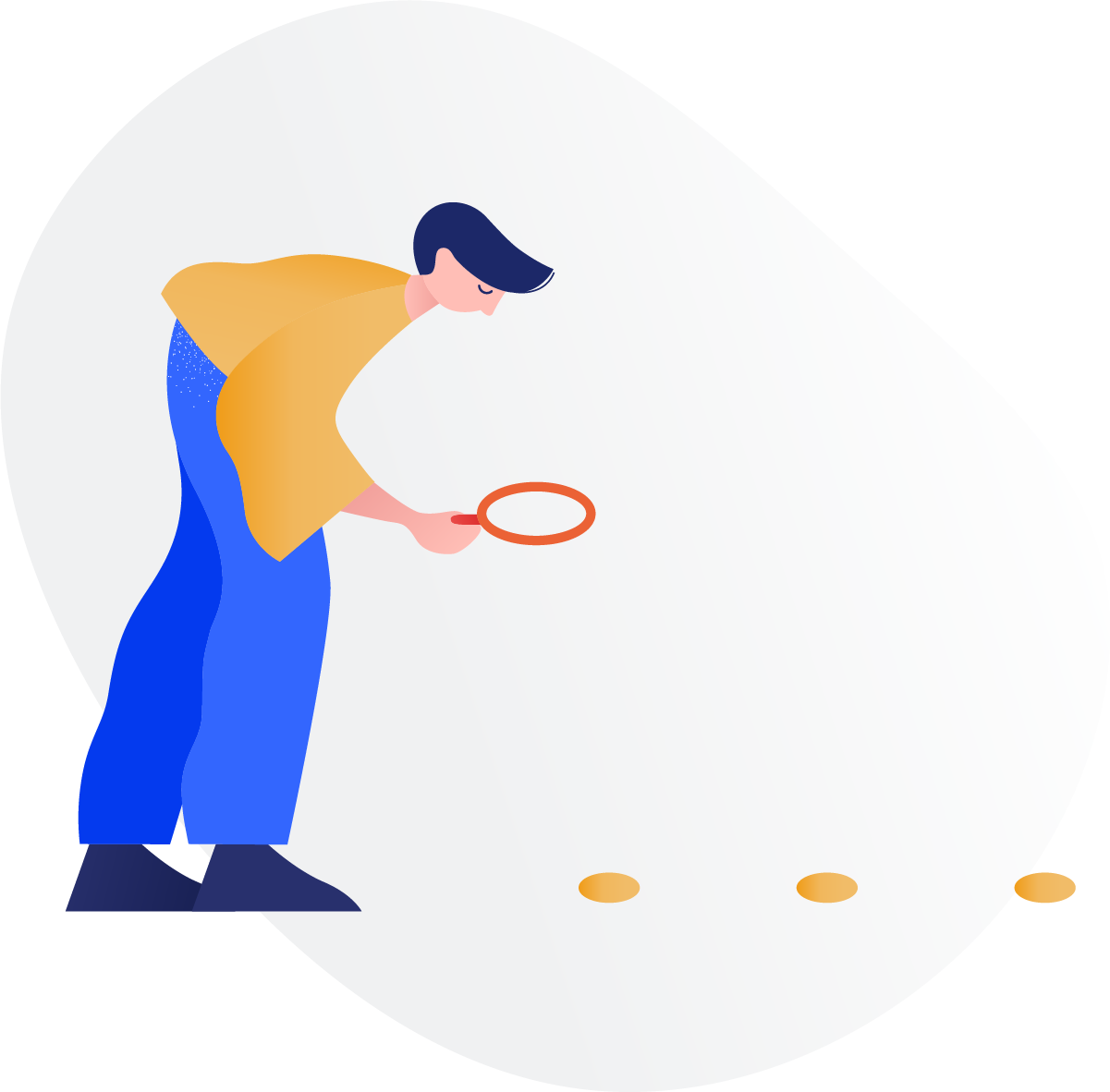
Oops! Something went wrong.
Looks like we were not able to place the reservation. Kindly try again later.
Are you sure you want to remove the book from the shelf?
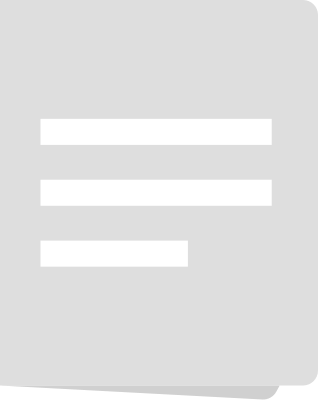
Approximate residual balancing
by
Wager, Stefan
, Athey, Susan
, Imbens, Guido W.
in
Adjustment
/ Assumptions
/ Averages
/ Causal inference
/ Inference
/ Linear models
/ Linearity
/ Parameters
/ Potential outcomes
/ Pretreatment
/ Propensity
/ Propensity score
/ Random variables
/ Regression analysis
/ Regression models
/ Sparse estimation
2018
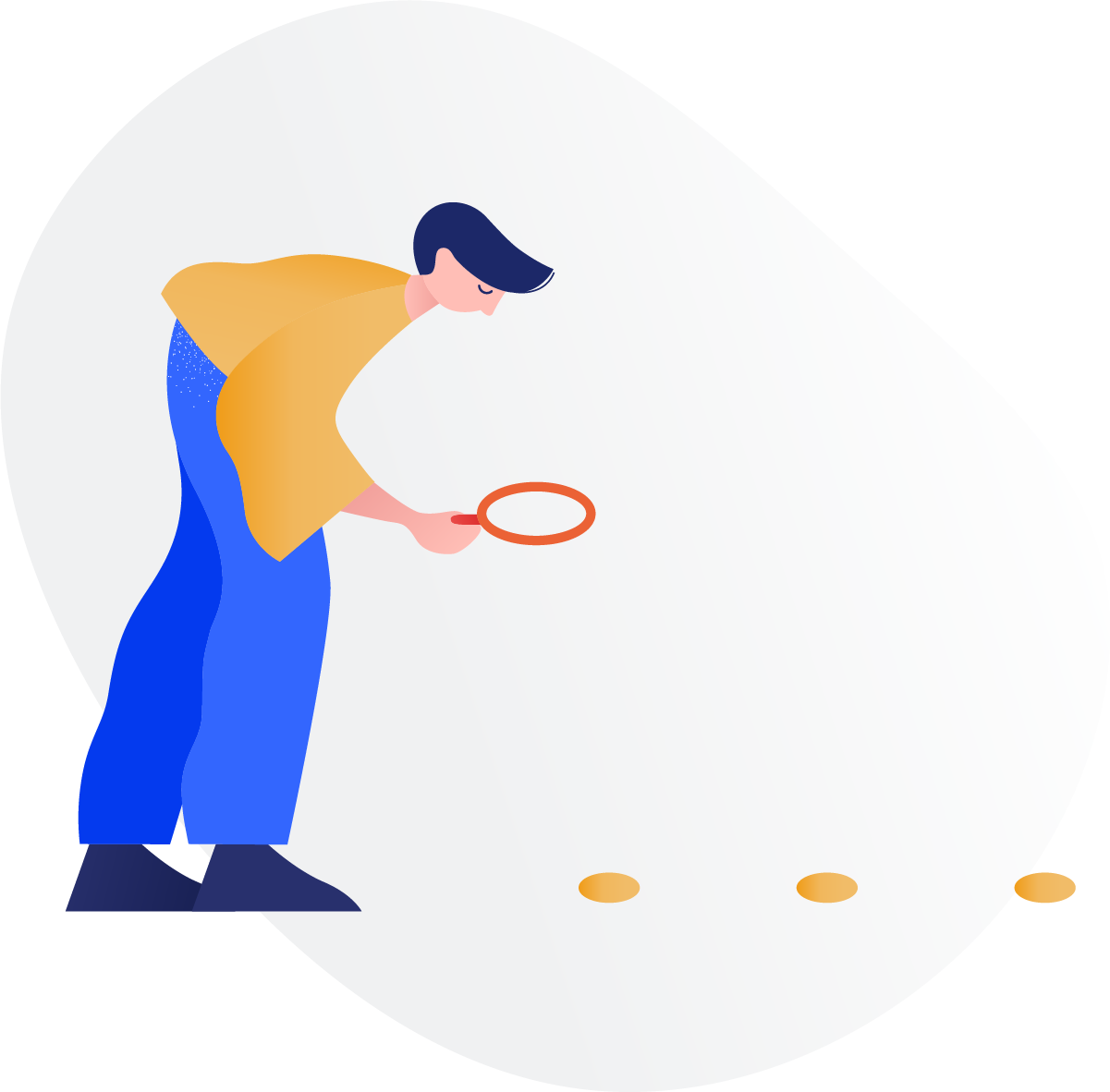
Oops! Something went wrong.
While trying to remove the title from your shelf something went wrong :( Kindly try again later!
Do you wish to request the book?
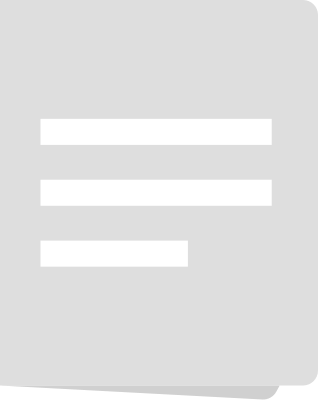
Approximate residual balancing
by
Wager, Stefan
, Athey, Susan
, Imbens, Guido W.
in
Adjustment
/ Assumptions
/ Averages
/ Causal inference
/ Inference
/ Linear models
/ Linearity
/ Parameters
/ Potential outcomes
/ Pretreatment
/ Propensity
/ Propensity score
/ Random variables
/ Regression analysis
/ Regression models
/ Sparse estimation
2018
Please be aware that the book you have requested cannot be checked out. If you would like to checkout this book, you can reserve another copy
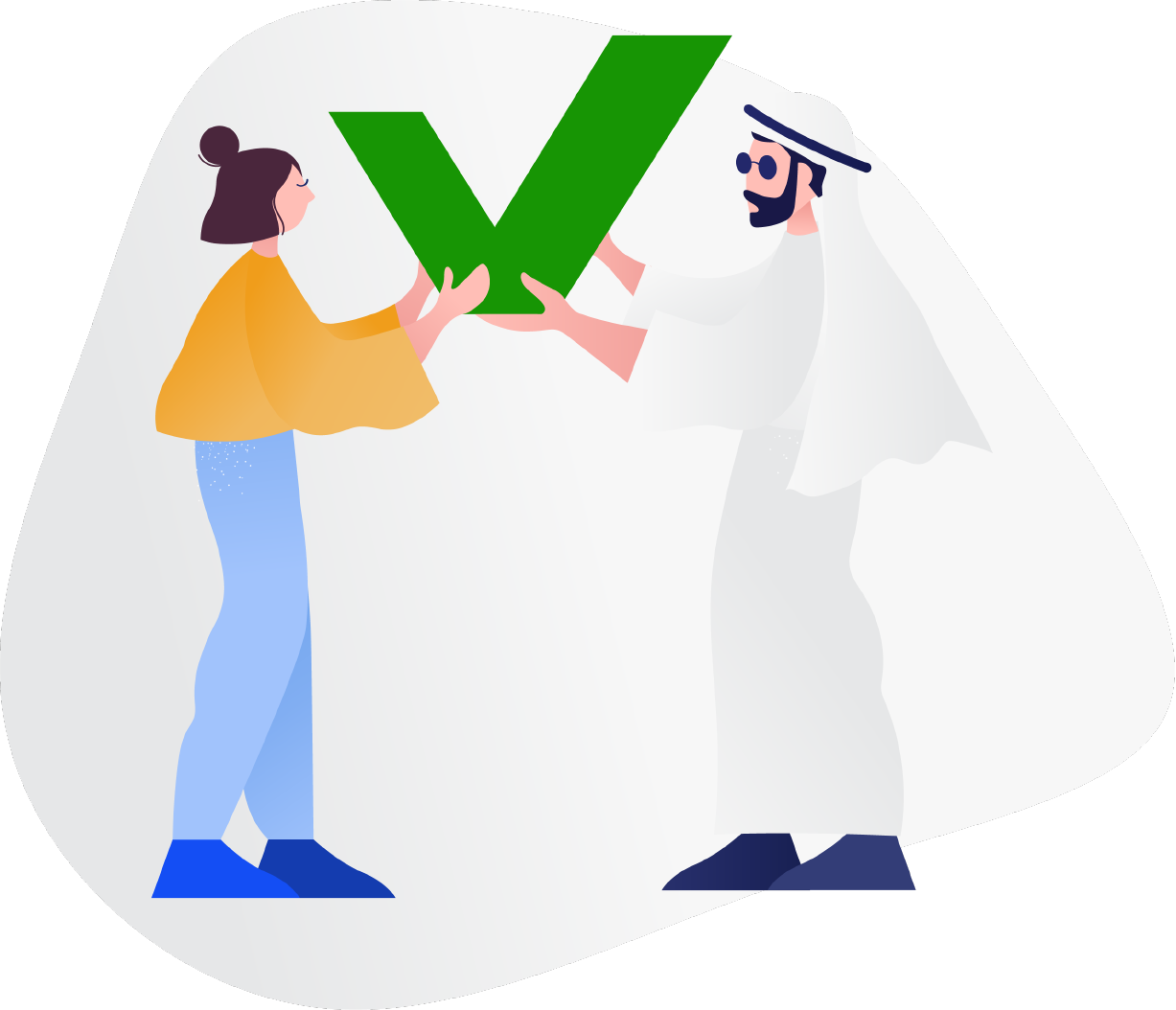
We have requested the book for you!
Your request is successful and it will be processed during the Library working hours. Please check the status of your request in My Requests.
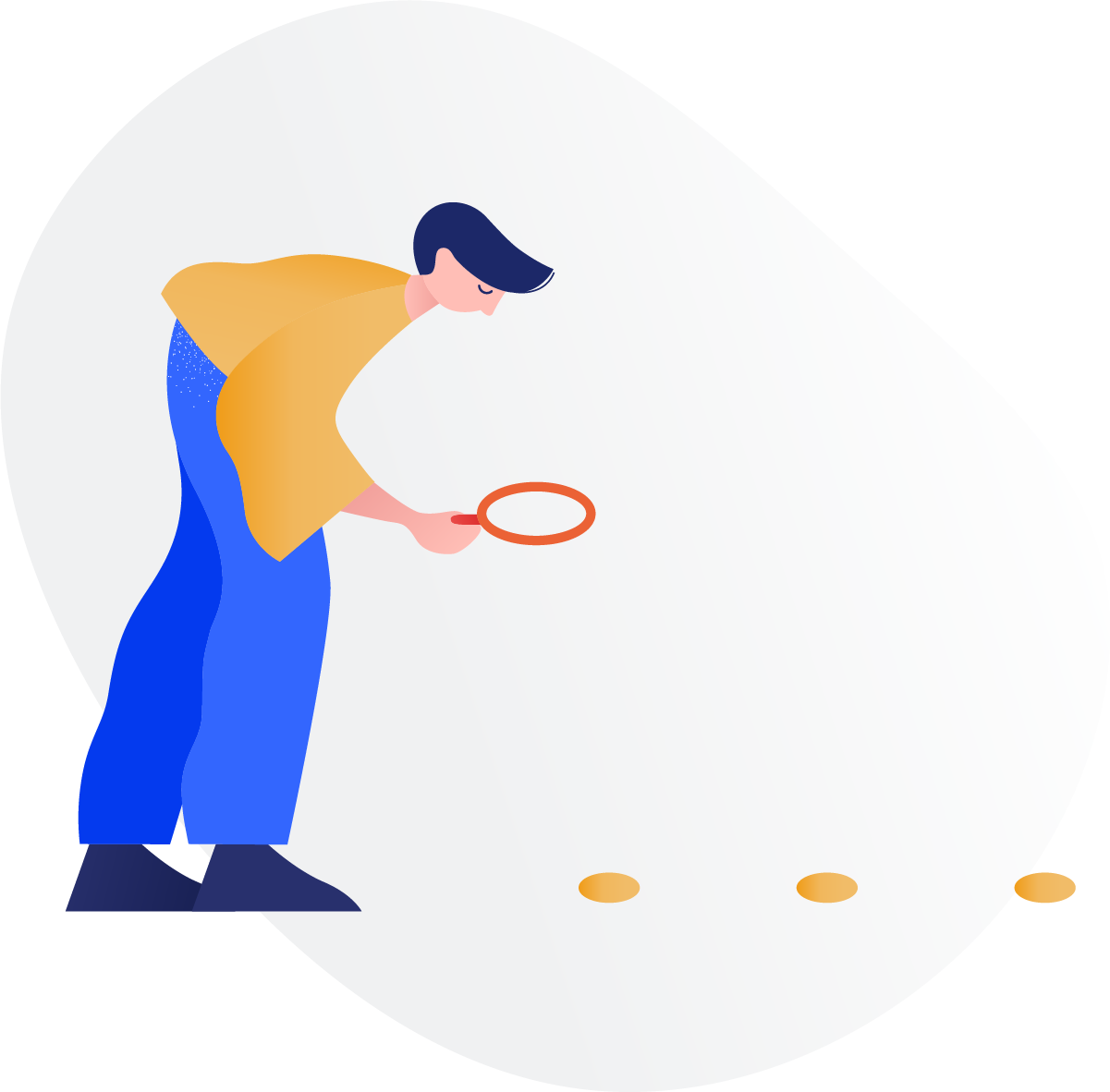
Oops! Something went wrong.
Looks like we were not able to place your request. Kindly try again later.

Journal Article
Approximate residual balancing
2018
Request now
and choose the collection method
Overview
There are many settings where researchers are interested in estimating average treatment effects and are willing to rely on the unconfoundedness assumption, which requires that the treatment assignment be as good as random conditional on pretreatment variables. The unconfoundedness assumption is often more plausible if a large number of pretreatment variables are included in the analysis, but this can worsen the performance of standard approaches to treatment effect estimation. We develop a method for debiasing penalized regression adjustments to allow sparse regression methods like the lasso to be used for √n-consistent inference of average treatment effects in high dimensional linear models. Given linearity, we do not need to assume that the treatment propensities are estimable, or that the average treatment effect is a sparse contrast of the outcome model parameters. Rather, in addition to standard assumptions used to make lasso regression on the outcome model consistent under 1-norm error, we require only overlap, i.e. that the propensity score be uniformly bounded away from 0 and 1. Procedurally, our method combines balancing weights with a regularized regression adjustment.
Publisher
Wiley,Blackwell Publishing Ltd
Subject
This website uses cookies to ensure you get the best experience on our website.