Asset Details
MbrlCatalogueTitleDetail
Do you wish to reserve the book?
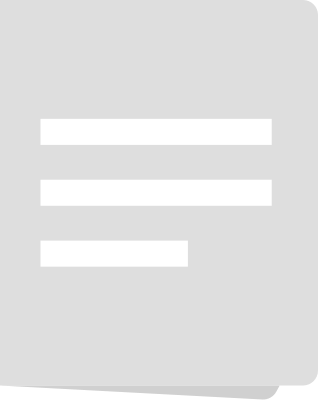
Big data stream analysis: a systematic literature review
by
Daramola, Olawande
, Kolajo, Taiwo
, Adebiyi, Ayodele
in
Algorithms
/ Analytics
/ Big Data
/ Big data stream analysis
/ Big data streaming tools and technologies
/ Communications Engineering
/ Computational Science and Engineering
/ Computer Science
/ Data analysis
/ Data management
/ Data mining
/ Data Mining and Knowledge Discovery
/ Data transmission
/ Database Management
/ Dynamic characteristics
/ Empirical analysis
/ Information Storage and Retrieval
/ Iterative methods
/ Literature reviews
/ Mathematical Applications in Computer Science
/ Networks
/ Parallel processing
/ Resource allocation
/ Stream computing
/ Survey Paper
2019
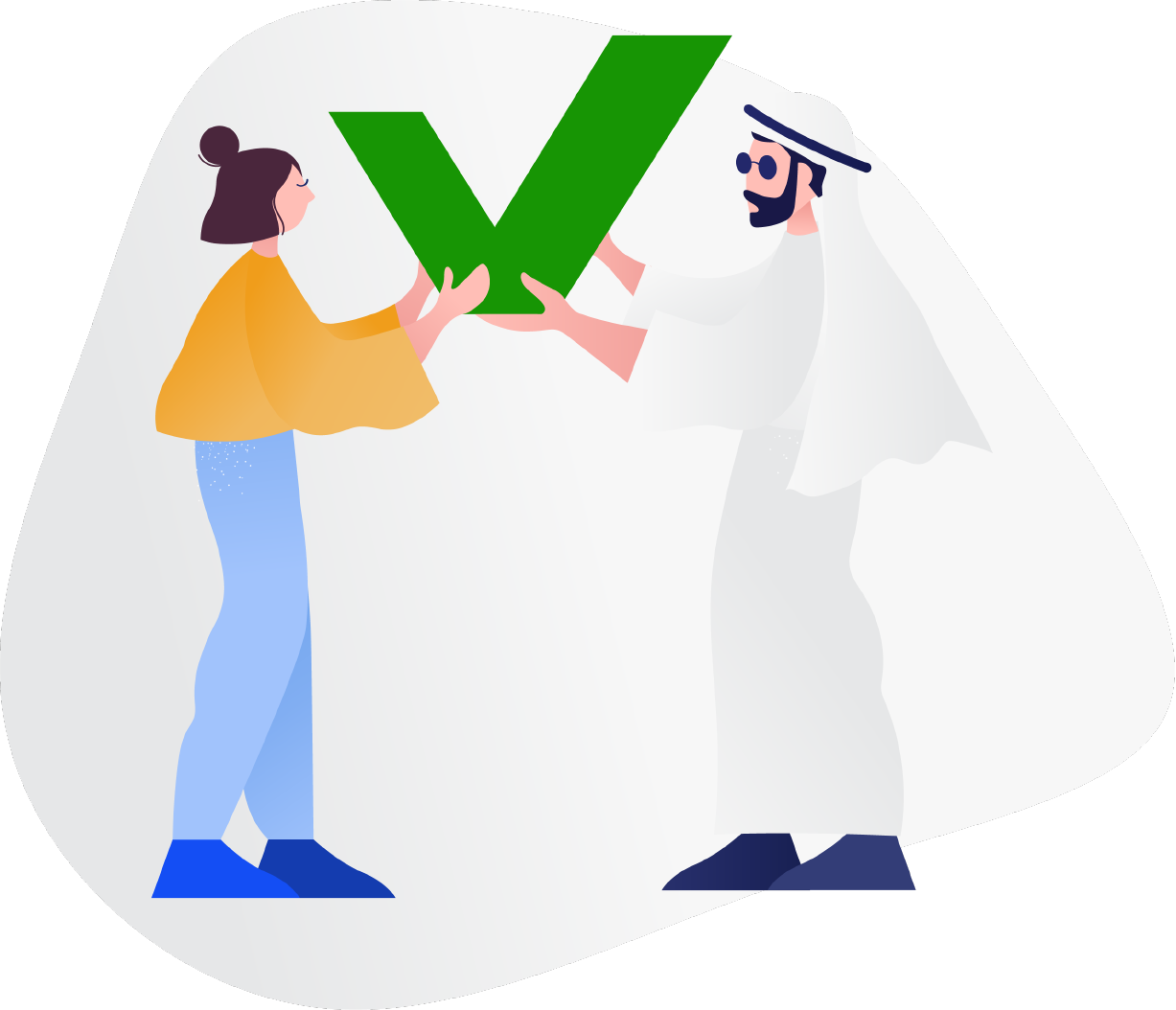
Hey, we have placed the reservation for you!
By the way, why not check out events that you can attend while you pick your title.
You are currently in the queue to collect this book. You will be notified once it is your turn to collect the book.
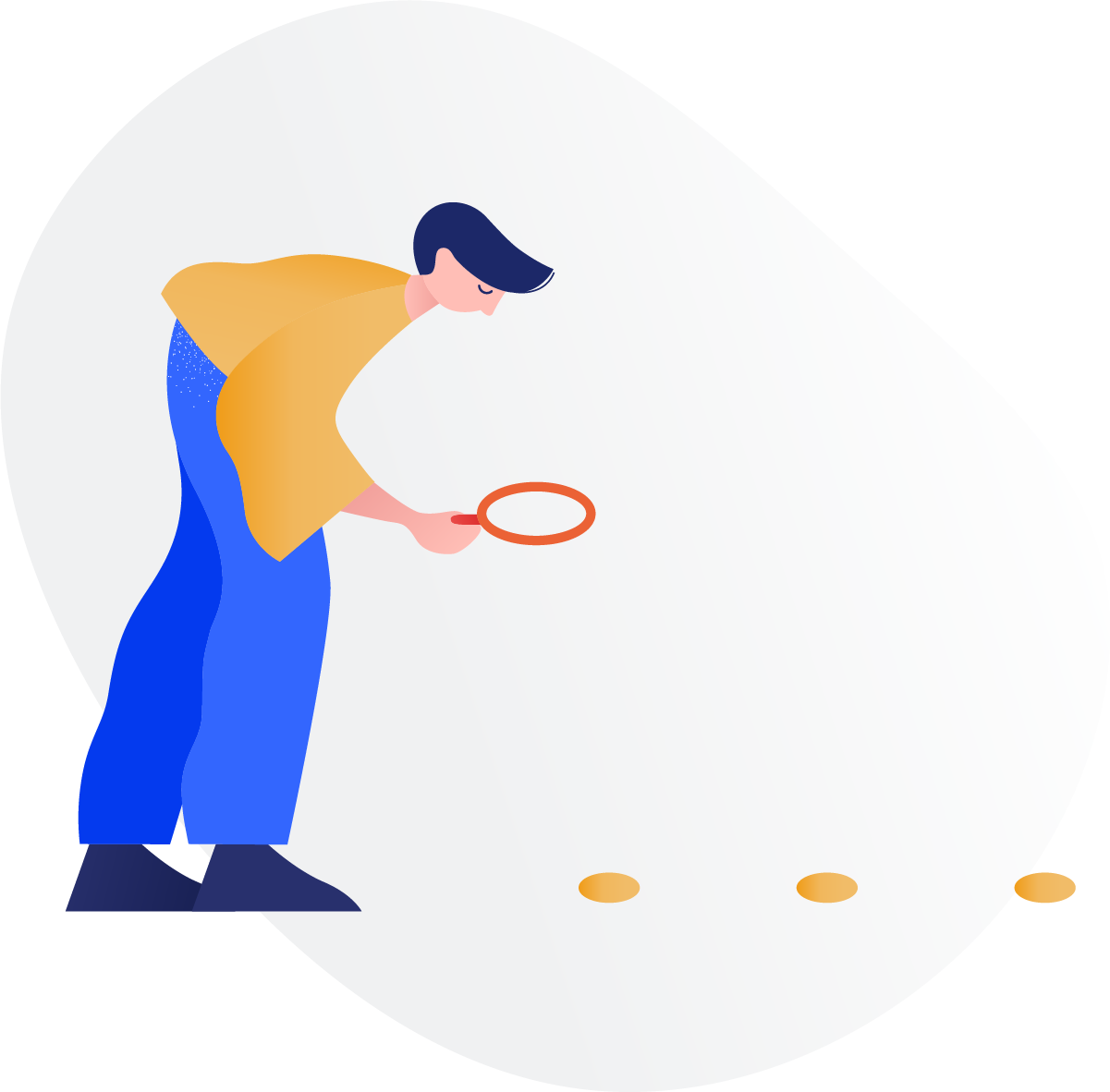
Oops! Something went wrong.
Looks like we were not able to place the reservation. Kindly try again later.
Are you sure you want to remove the book from the shelf?
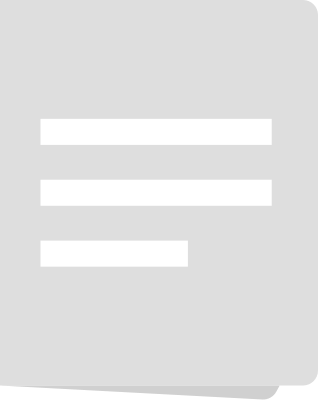
Big data stream analysis: a systematic literature review
by
Daramola, Olawande
, Kolajo, Taiwo
, Adebiyi, Ayodele
in
Algorithms
/ Analytics
/ Big Data
/ Big data stream analysis
/ Big data streaming tools and technologies
/ Communications Engineering
/ Computational Science and Engineering
/ Computer Science
/ Data analysis
/ Data management
/ Data mining
/ Data Mining and Knowledge Discovery
/ Data transmission
/ Database Management
/ Dynamic characteristics
/ Empirical analysis
/ Information Storage and Retrieval
/ Iterative methods
/ Literature reviews
/ Mathematical Applications in Computer Science
/ Networks
/ Parallel processing
/ Resource allocation
/ Stream computing
/ Survey Paper
2019
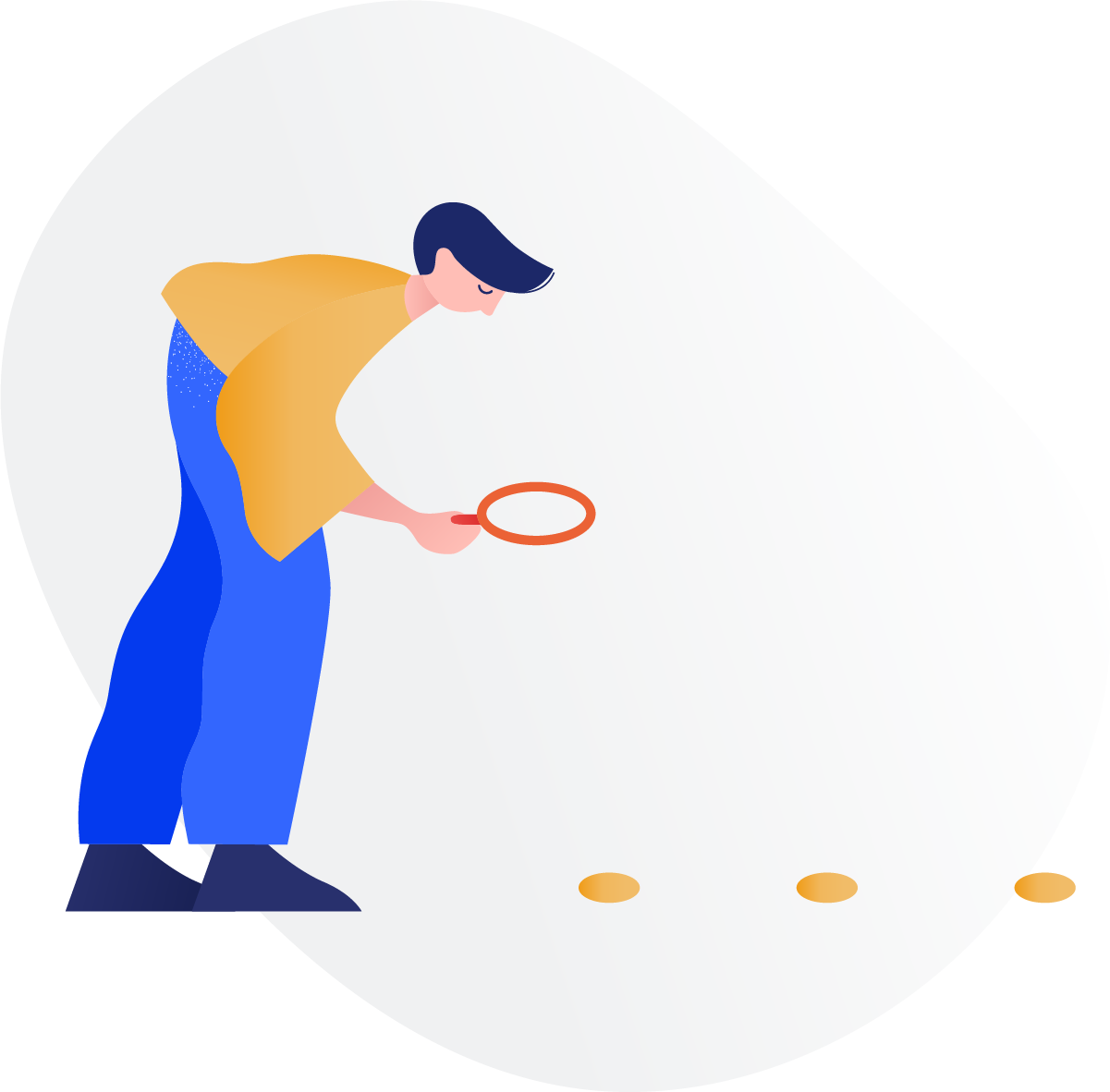
Oops! Something went wrong.
While trying to remove the title from your shelf something went wrong :( Kindly try again later!
Do you wish to request the book?
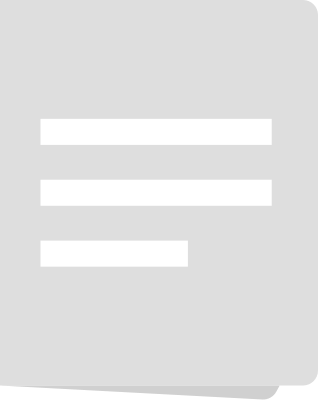
Big data stream analysis: a systematic literature review
by
Daramola, Olawande
, Kolajo, Taiwo
, Adebiyi, Ayodele
in
Algorithms
/ Analytics
/ Big Data
/ Big data stream analysis
/ Big data streaming tools and technologies
/ Communications Engineering
/ Computational Science and Engineering
/ Computer Science
/ Data analysis
/ Data management
/ Data mining
/ Data Mining and Knowledge Discovery
/ Data transmission
/ Database Management
/ Dynamic characteristics
/ Empirical analysis
/ Information Storage and Retrieval
/ Iterative methods
/ Literature reviews
/ Mathematical Applications in Computer Science
/ Networks
/ Parallel processing
/ Resource allocation
/ Stream computing
/ Survey Paper
2019
Please be aware that the book you have requested cannot be checked out. If you would like to checkout this book, you can reserve another copy
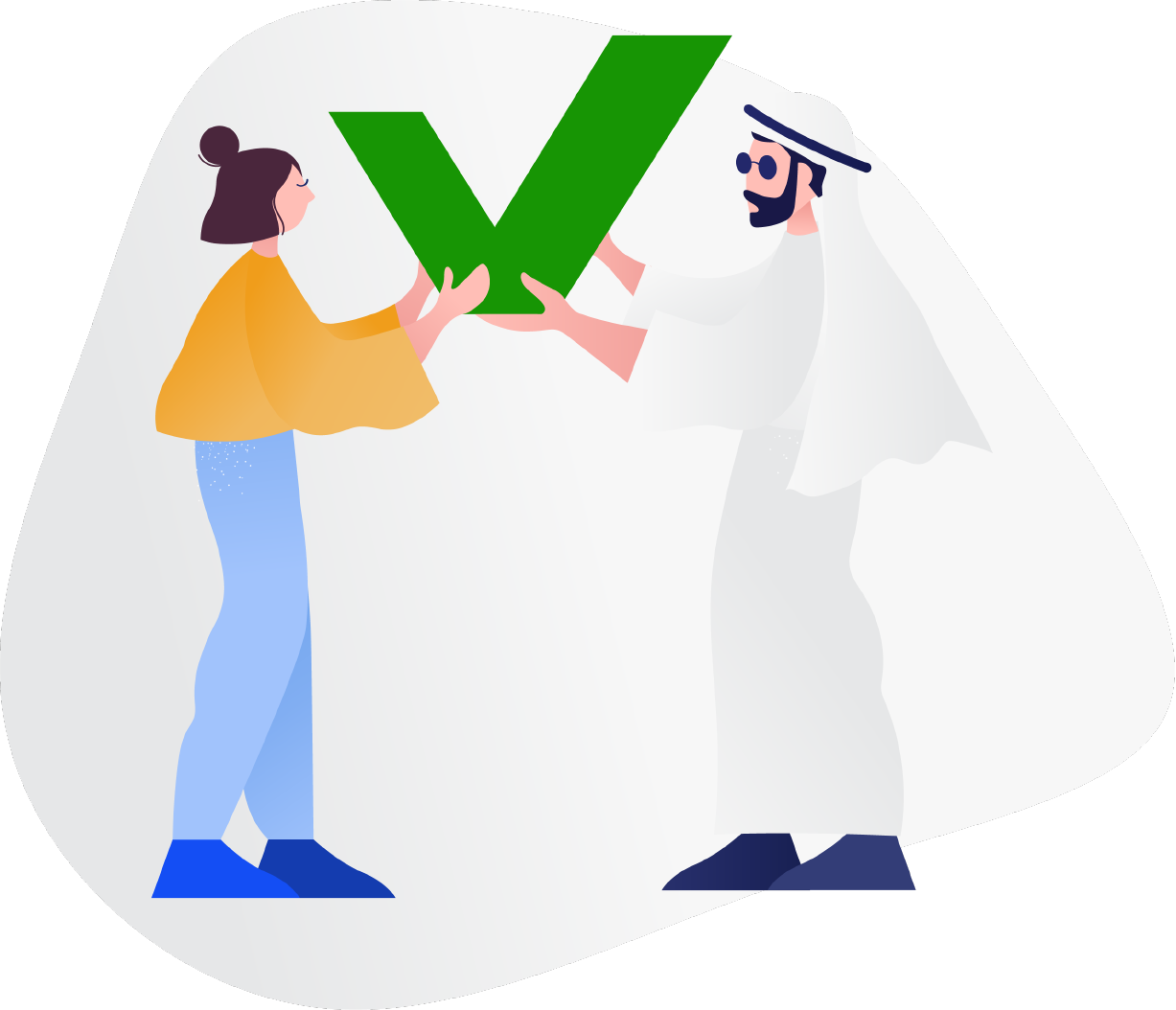
We have requested the book for you!
Your request is successful and it will be processed during the Library working hours. Please check the status of your request in My Requests.
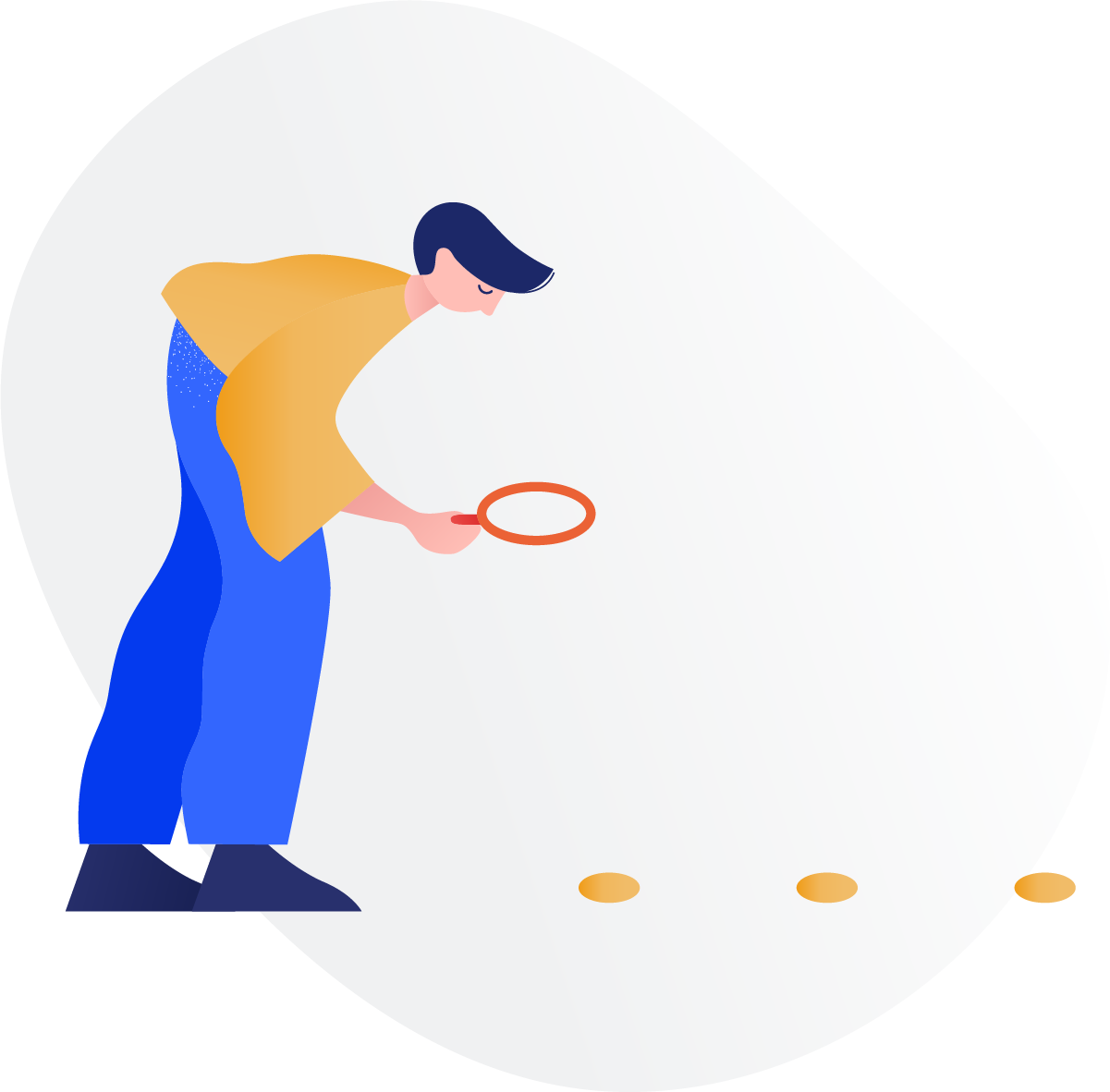
Oops! Something went wrong.
Looks like we were not able to place your request. Kindly try again later.

Journal Article
Big data stream analysis: a systematic literature review
2019
Request now
and choose the collection method
Overview
Recently, big data streams have become ubiquitous due to the fact that a number of applications generate a huge amount of data at a great velocity. This made it difficult for existing data mining tools, technologies, methods, and techniques to be applied directly on big data streams due to the inherent dynamic characteristics of big data. In this paper, a systematic review of big data streams analysis which employed a rigorous and methodical approach to look at the trends of big data stream tools and technologies as well as methods and techniques employed in analysing big data streams. It provides a global view of big data stream tools and technologies and its comparisons. Three major databases, Scopus, ScienceDirect and EBSCO, which indexes journals and conferences that are promoted by entities such as IEEE, ACM, SpringerLink, and Elsevier were explored as data sources. Out of the initial 2295 papers that resulted from the first search string, 47 papers were found to be relevant to our research questions after implementing the inclusion and exclusion criteria. The study found that scalability, privacy and load balancing issues as well as empirical analysis of big data streams and technologies are still open for further research efforts. We also found that although, significant research efforts have been directed to real-time analysis of big data stream not much attention has been given to the preprocessing stage of big data streams. Only a few big data streaming tools and technologies can do all of the batch, streaming, and iterative jobs; there seems to be no big data tool and technology that offers all the key features required for now and standard benchmark dataset for big data streaming analytics has not been widely adopted. In conclusion, it was recommended that research efforts should be geared towards developing scalable frameworks and algorithms that will accommodate data stream computing mode, effective resource allocation strategy and parallelization issues to cope with the ever-growing size and complexity of data.
Publisher
Springer International Publishing,Springer Nature B.V,SpringerOpen
This website uses cookies to ensure you get the best experience on our website.