Asset Details
MbrlCatalogueTitleDetail
Do you wish to reserve the book?
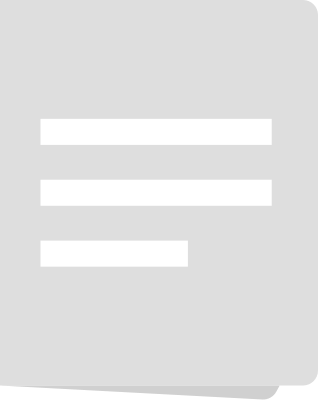
Evaluating EMG Feature and Classifier Selection for Application to Partial-Hand Prosthesis Control
by
Hargrove, Levi J
, Adewuyi, Adenike A
, Kuiken, Todd A
in
Amputation
/ Classification
/ Control algorithms
/ Electrodes
/ Electromyography
/ electromyography (EMG)
/ Feature Selection
/ Hand
/ intrinsic hand muscles
/ myoelectric control
/ Neural networks
/ Neuroscience
/ partial-hand amputee
/ Pattern recognition
/ Pattern recognition systems
/ Prostheses
/ Prosthetics
/ Studies
/ Wrist
2016
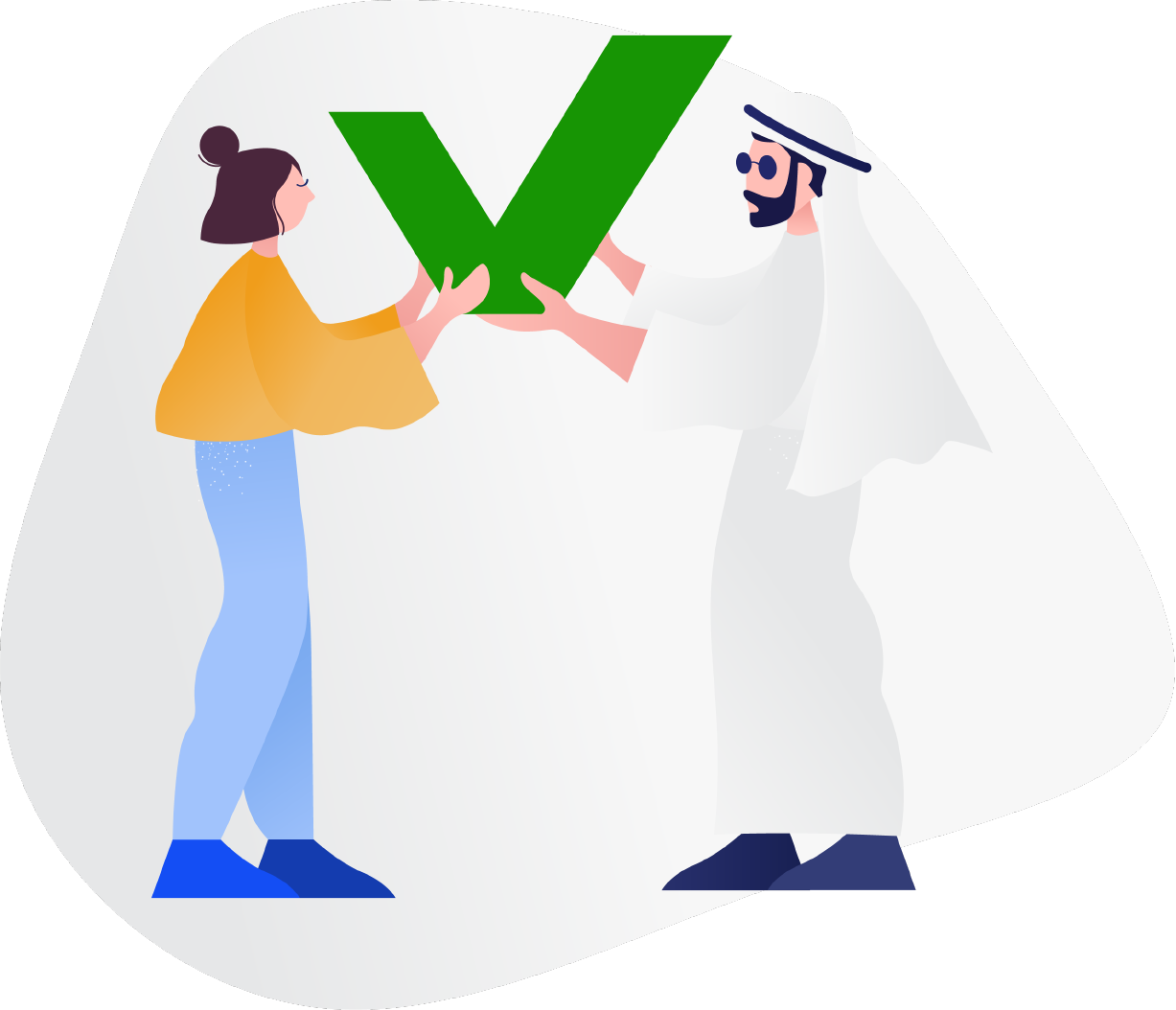
Hey, we have placed the reservation for you!
By the way, why not check out events that you can attend while you pick your title.
You are currently in the queue to collect this book. You will be notified once it is your turn to collect the book.
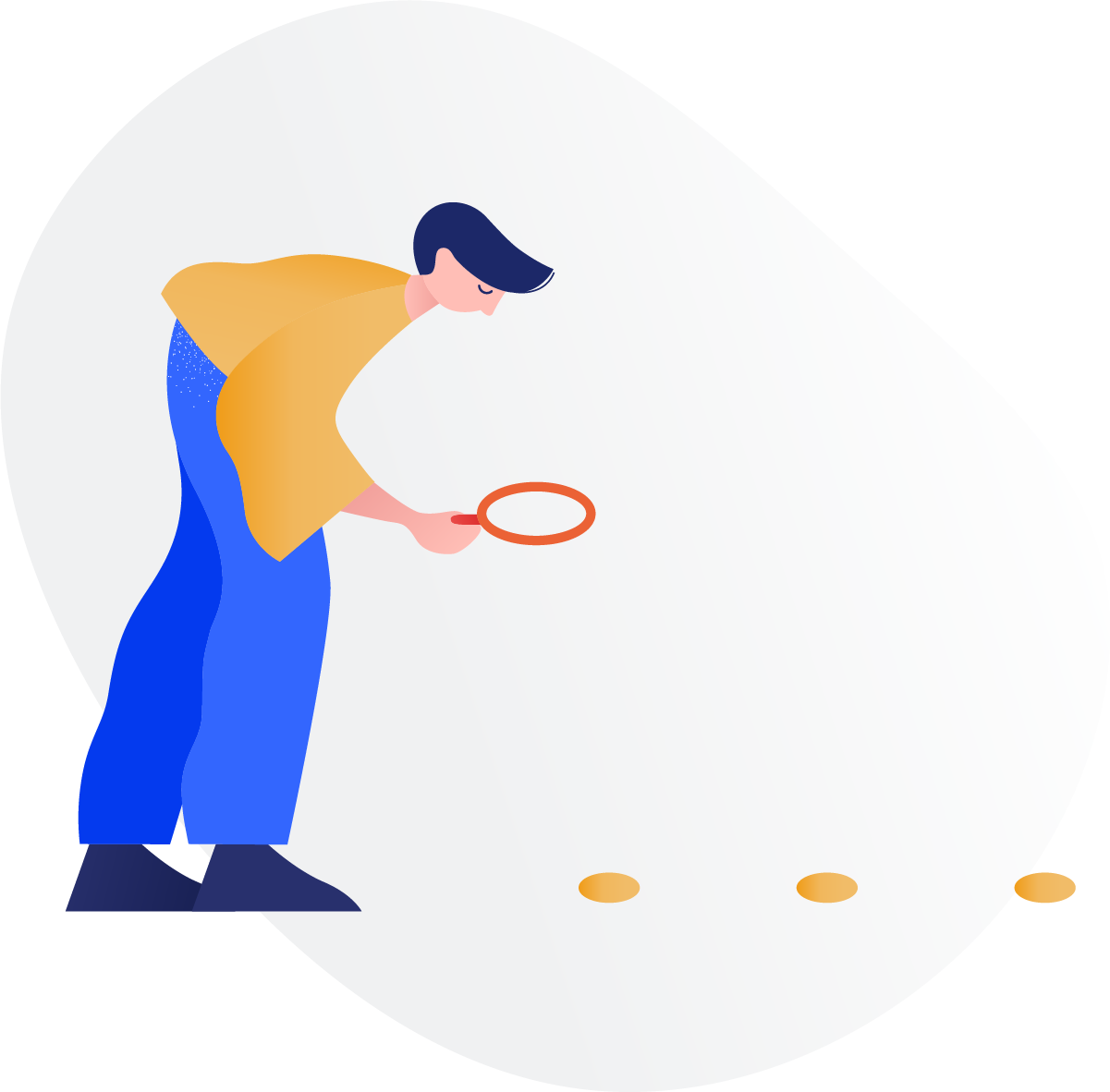
Oops! Something went wrong.
Looks like we were not able to place the reservation. Kindly try again later.
Are you sure you want to remove the book from the shelf?
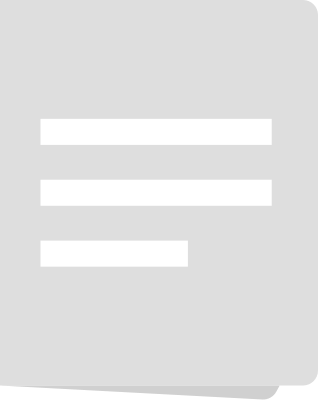
Evaluating EMG Feature and Classifier Selection for Application to Partial-Hand Prosthesis Control
by
Hargrove, Levi J
, Adewuyi, Adenike A
, Kuiken, Todd A
in
Amputation
/ Classification
/ Control algorithms
/ Electrodes
/ Electromyography
/ electromyography (EMG)
/ Feature Selection
/ Hand
/ intrinsic hand muscles
/ myoelectric control
/ Neural networks
/ Neuroscience
/ partial-hand amputee
/ Pattern recognition
/ Pattern recognition systems
/ Prostheses
/ Prosthetics
/ Studies
/ Wrist
2016
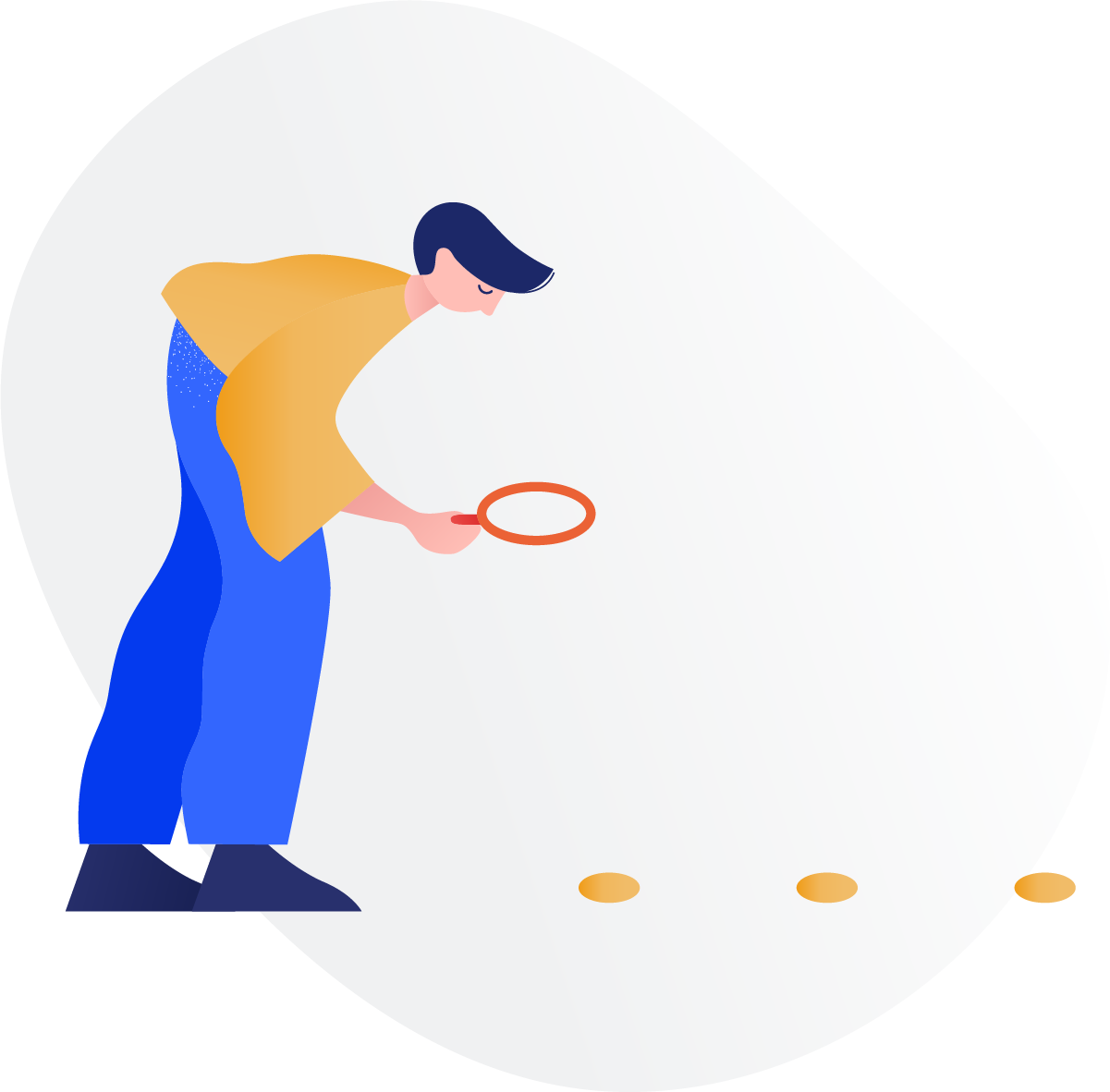
Oops! Something went wrong.
While trying to remove the title from your shelf something went wrong :( Kindly try again later!
Do you wish to request the book?
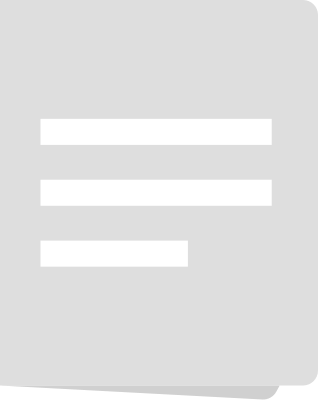
Evaluating EMG Feature and Classifier Selection for Application to Partial-Hand Prosthesis Control
by
Hargrove, Levi J
, Adewuyi, Adenike A
, Kuiken, Todd A
in
Amputation
/ Classification
/ Control algorithms
/ Electrodes
/ Electromyography
/ electromyography (EMG)
/ Feature Selection
/ Hand
/ intrinsic hand muscles
/ myoelectric control
/ Neural networks
/ Neuroscience
/ partial-hand amputee
/ Pattern recognition
/ Pattern recognition systems
/ Prostheses
/ Prosthetics
/ Studies
/ Wrist
2016
Please be aware that the book you have requested cannot be checked out. If you would like to checkout this book, you can reserve another copy
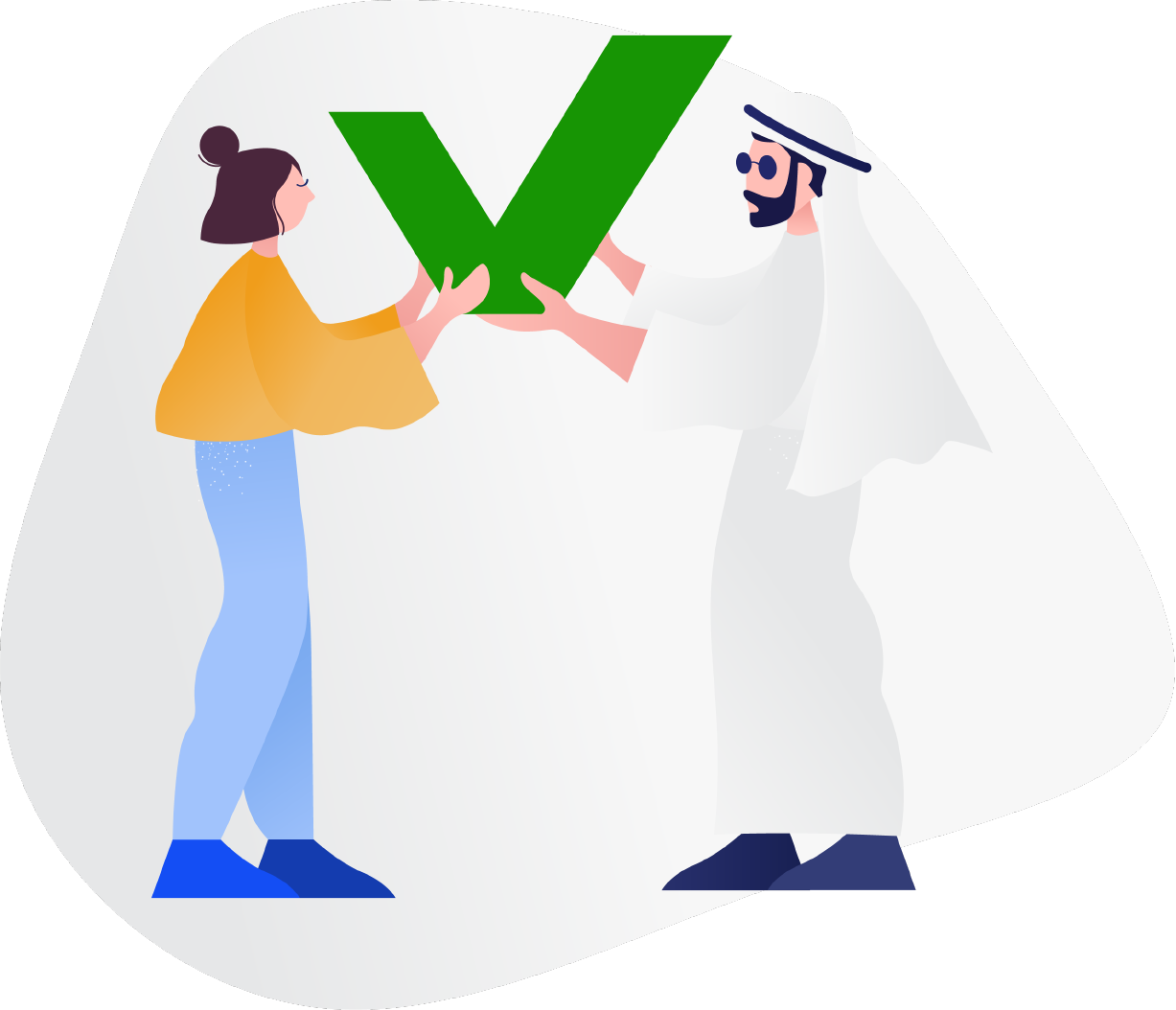
We have requested the book for you!
Your request is successful and it will be processed during the Library working hours. Please check the status of your request in My Requests.
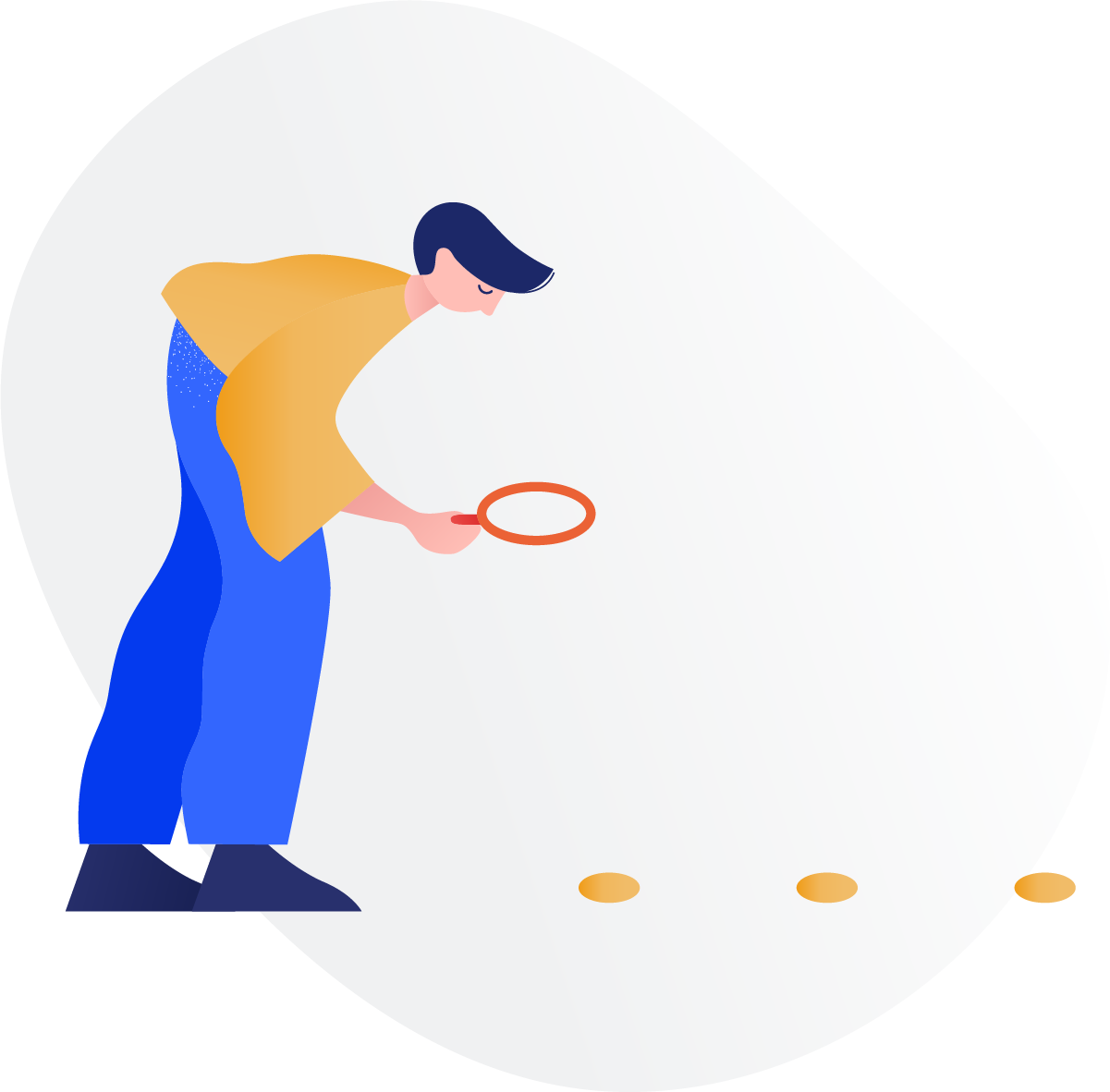
Oops! Something went wrong.
Looks like we were not able to place your request. Kindly try again later.
Evaluating EMG Feature and Classifier Selection for Application to Partial-Hand Prosthesis Control

Journal Article
Evaluating EMG Feature and Classifier Selection for Application to Partial-Hand Prosthesis Control
2016
Request now
and choose the collection method
Overview
Pattern recognition-based myoelectric control of upper-limb prostheses has the potential to restore control of multiple degrees of freedom. Though this control method has been extensively studied in individuals with higher-level amputations, few studies have investigated its effectiveness for individuals with partial-hand amputations. Most partial-hand amputees retain a functional wrist and the ability of pattern recognition-based methods to correctly classify hand motions from different wrist positions is not well studied. In this study, focusing on partial-hand amputees, we evaluate (1) the performance of non-linear and linear pattern recognition algorithms and (2) the performance of optimal EMG feature subsets for classification of four hand motion classes in different wrist positions for 16 non-amputees and 4 amputees. Our results show that linear discriminant analysis and linear and non-linear artificial neural networks perform significantly better than the quadratic discriminant analysis for both non-amputees and partial-hand amputees. For amputees, including information from multiple wrist positions significantly decreased error (
< 0.001) but no further significant decrease in error occurred when more than 4, 2, or 3 positions were included for the extrinsic (
= 0.07), intrinsic (
= 0.06), or combined extrinsic and intrinsic muscle EMG (
= 0.08), respectively. Finally, we found that a feature set determined by selecting optimal features from each channel outperformed the commonly used time domain (
< 0.001) and time domain/autoregressive feature sets (
< 0.01). This method can be used as a screening filter to select the features from each channel that provide the best classification of hand postures across different wrist positions.
This website uses cookies to ensure you get the best experience on our website.