Asset Details
MbrlCatalogueTitleDetail
Do you wish to reserve the book?
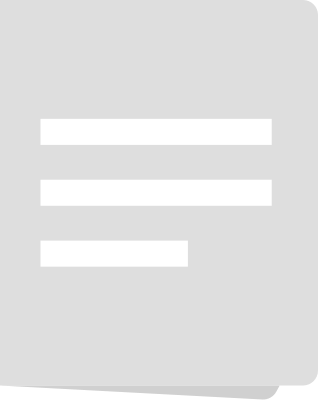
Generating synthetic gait patterns based on benchmark datasets for controlling prosthetic legs
by
Kim, Minjae
, Hargrove, Levi J
in
Amputation
/ Analysis
/ Ankle
/ Artificial legs
/ Artificial neural networks
/ Benchmark data
/ Benchmarks
/ Climbing
/ Control methods
/ Control systems
/ Controllers
/ Damping
/ Datasets
/ Electromyography
/ Engineering research
/ Gait
/ Generative adversarial network
/ Generative adversarial networks
/ Impedance
/ Impedance control
/ Implants, Artificial
/ Kinematics
/ Leg
/ Legs
/ Liquors
/ Locomotion
/ Machine learning
/ Mathematical models
/ Mechanical properties
/ Neural networks
/ Parameters
/ Prostheses
/ Prosthesis
/ Self-help devices for the disabled
/ Sensors
/ Smartphones
/ Synthetic impedance parameters
/ Thigh
/ Training
/ Vertical loads
/ Walking
2023
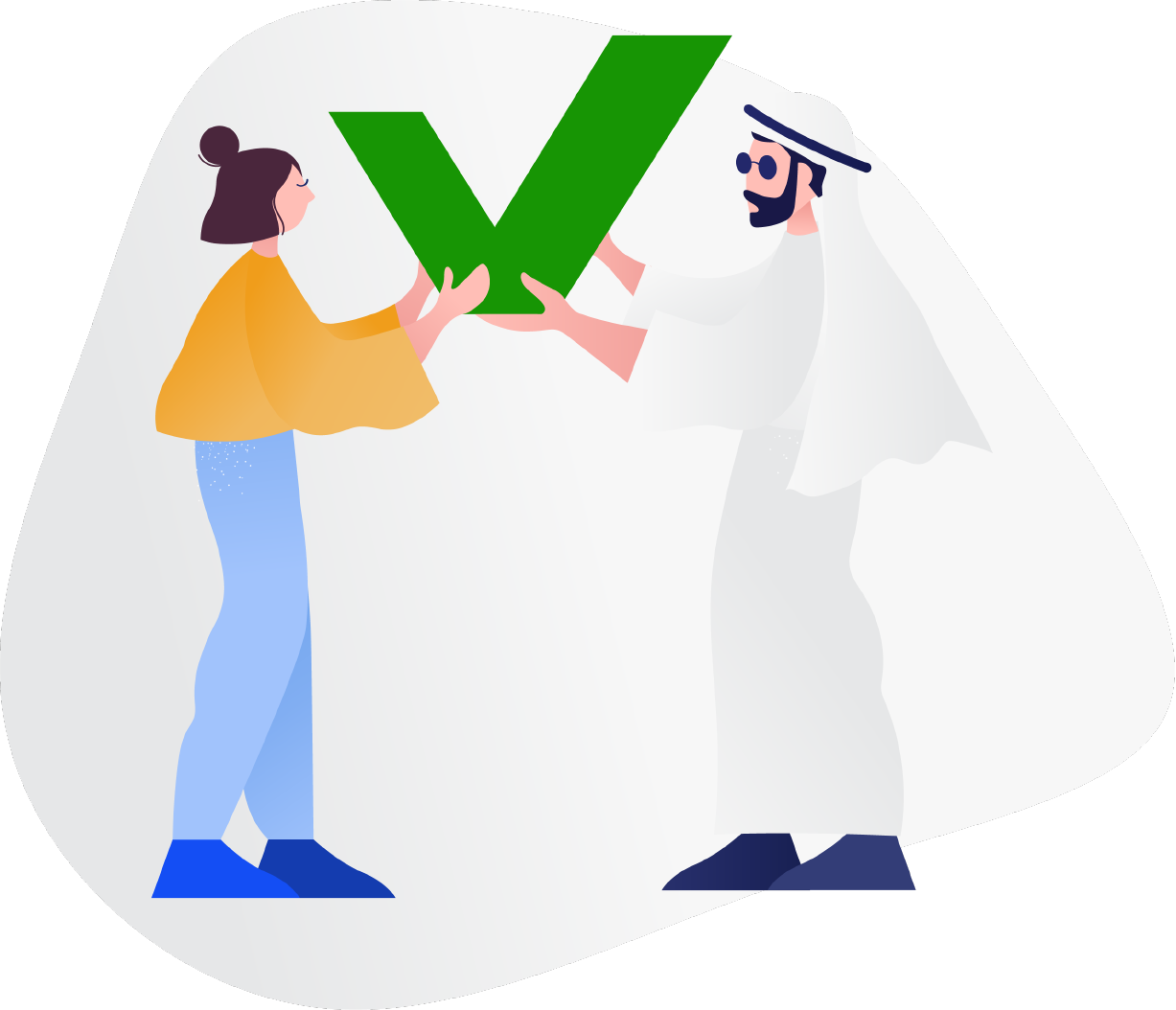
Hey, we have placed the reservation for you!
By the way, why not check out events that you can attend while you pick your title.
You are currently in the queue to collect this book. You will be notified once it is your turn to collect the book.
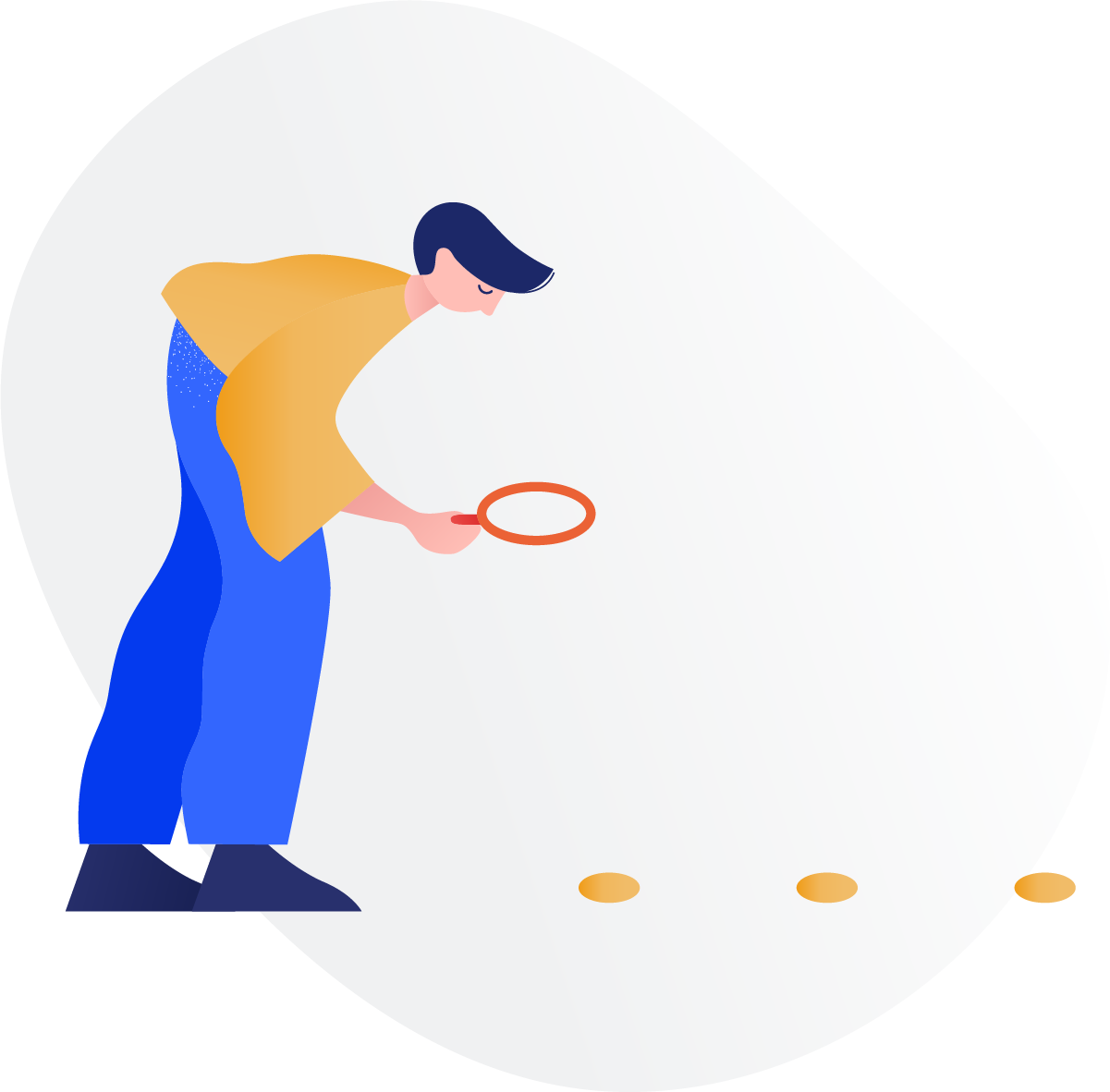
Oops! Something went wrong.
Looks like we were not able to place the reservation. Kindly try again later.
Are you sure you want to remove the book from the shelf?
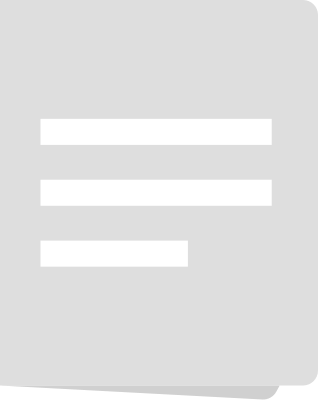
Generating synthetic gait patterns based on benchmark datasets for controlling prosthetic legs
by
Kim, Minjae
, Hargrove, Levi J
in
Amputation
/ Analysis
/ Ankle
/ Artificial legs
/ Artificial neural networks
/ Benchmark data
/ Benchmarks
/ Climbing
/ Control methods
/ Control systems
/ Controllers
/ Damping
/ Datasets
/ Electromyography
/ Engineering research
/ Gait
/ Generative adversarial network
/ Generative adversarial networks
/ Impedance
/ Impedance control
/ Implants, Artificial
/ Kinematics
/ Leg
/ Legs
/ Liquors
/ Locomotion
/ Machine learning
/ Mathematical models
/ Mechanical properties
/ Neural networks
/ Parameters
/ Prostheses
/ Prosthesis
/ Self-help devices for the disabled
/ Sensors
/ Smartphones
/ Synthetic impedance parameters
/ Thigh
/ Training
/ Vertical loads
/ Walking
2023
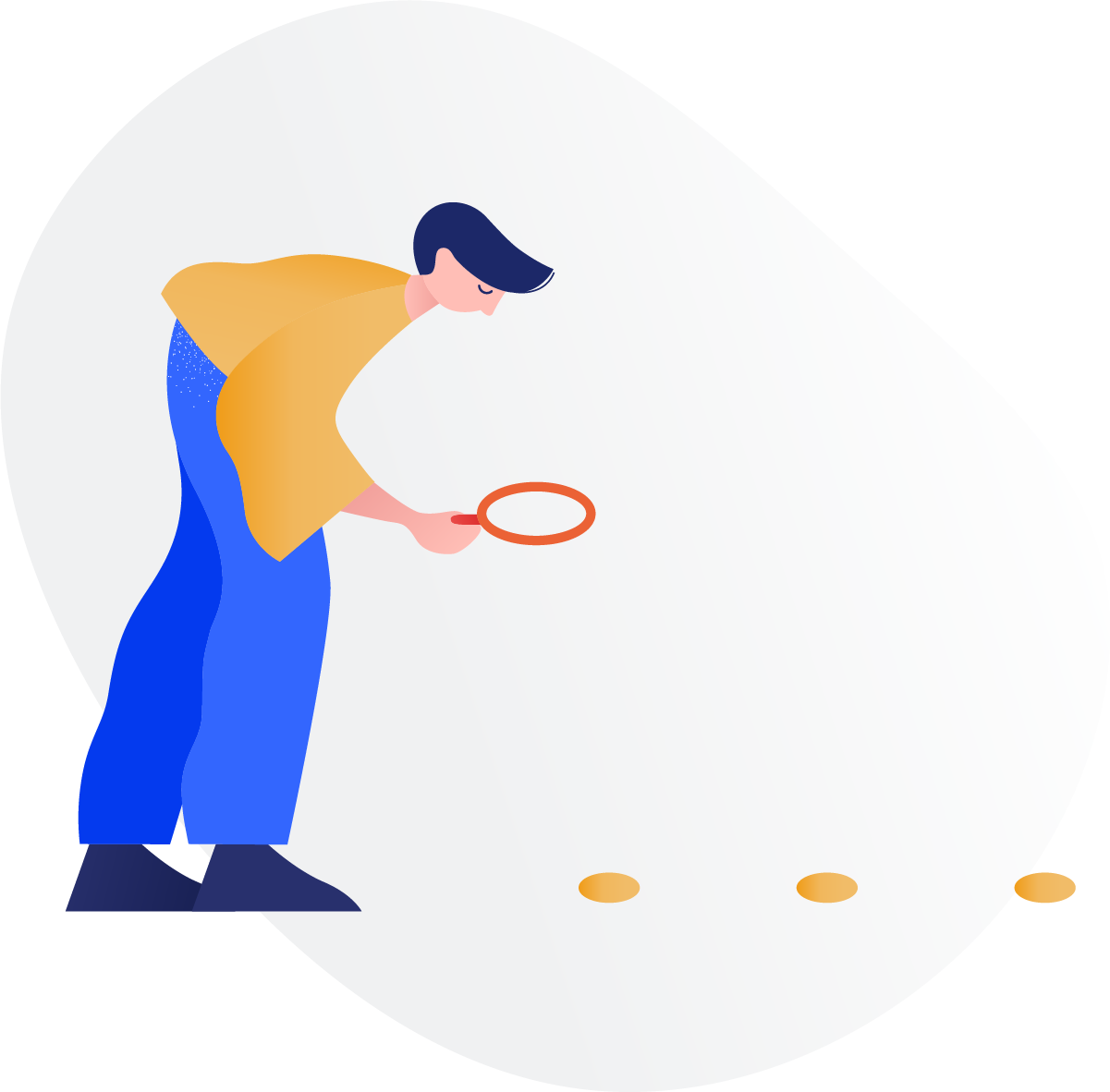
Oops! Something went wrong.
While trying to remove the title from your shelf something went wrong :( Kindly try again later!
Do you wish to request the book?
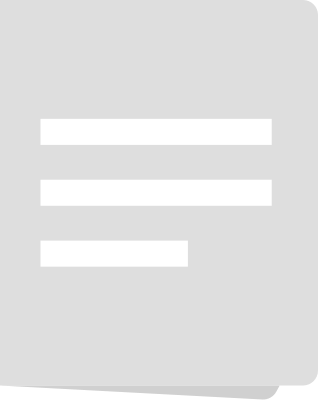
Generating synthetic gait patterns based on benchmark datasets for controlling prosthetic legs
by
Kim, Minjae
, Hargrove, Levi J
in
Amputation
/ Analysis
/ Ankle
/ Artificial legs
/ Artificial neural networks
/ Benchmark data
/ Benchmarks
/ Climbing
/ Control methods
/ Control systems
/ Controllers
/ Damping
/ Datasets
/ Electromyography
/ Engineering research
/ Gait
/ Generative adversarial network
/ Generative adversarial networks
/ Impedance
/ Impedance control
/ Implants, Artificial
/ Kinematics
/ Leg
/ Legs
/ Liquors
/ Locomotion
/ Machine learning
/ Mathematical models
/ Mechanical properties
/ Neural networks
/ Parameters
/ Prostheses
/ Prosthesis
/ Self-help devices for the disabled
/ Sensors
/ Smartphones
/ Synthetic impedance parameters
/ Thigh
/ Training
/ Vertical loads
/ Walking
2023
Please be aware that the book you have requested cannot be checked out. If you would like to checkout this book, you can reserve another copy
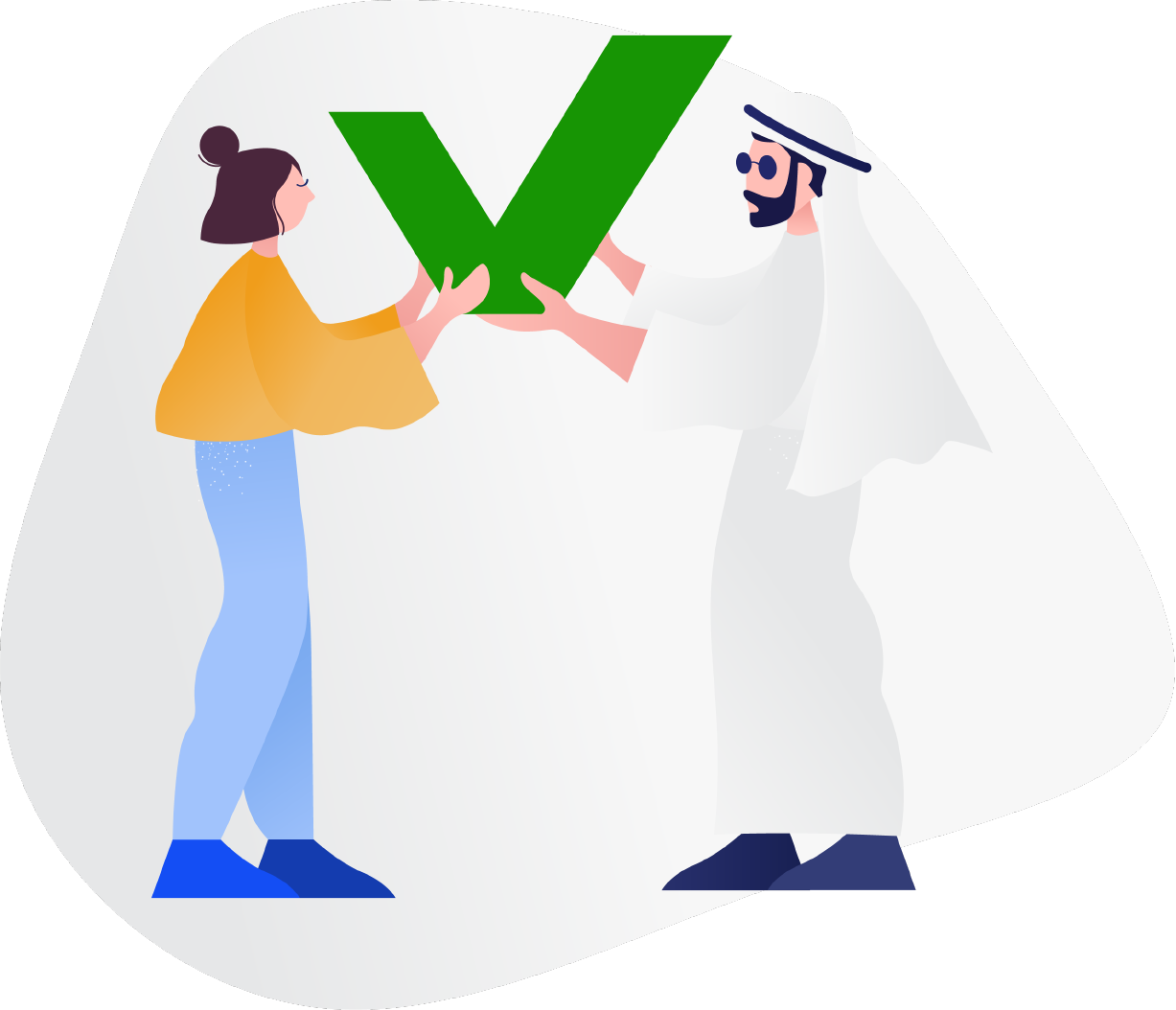
We have requested the book for you!
Your request is successful and it will be processed during the Library working hours. Please check the status of your request in My Requests.
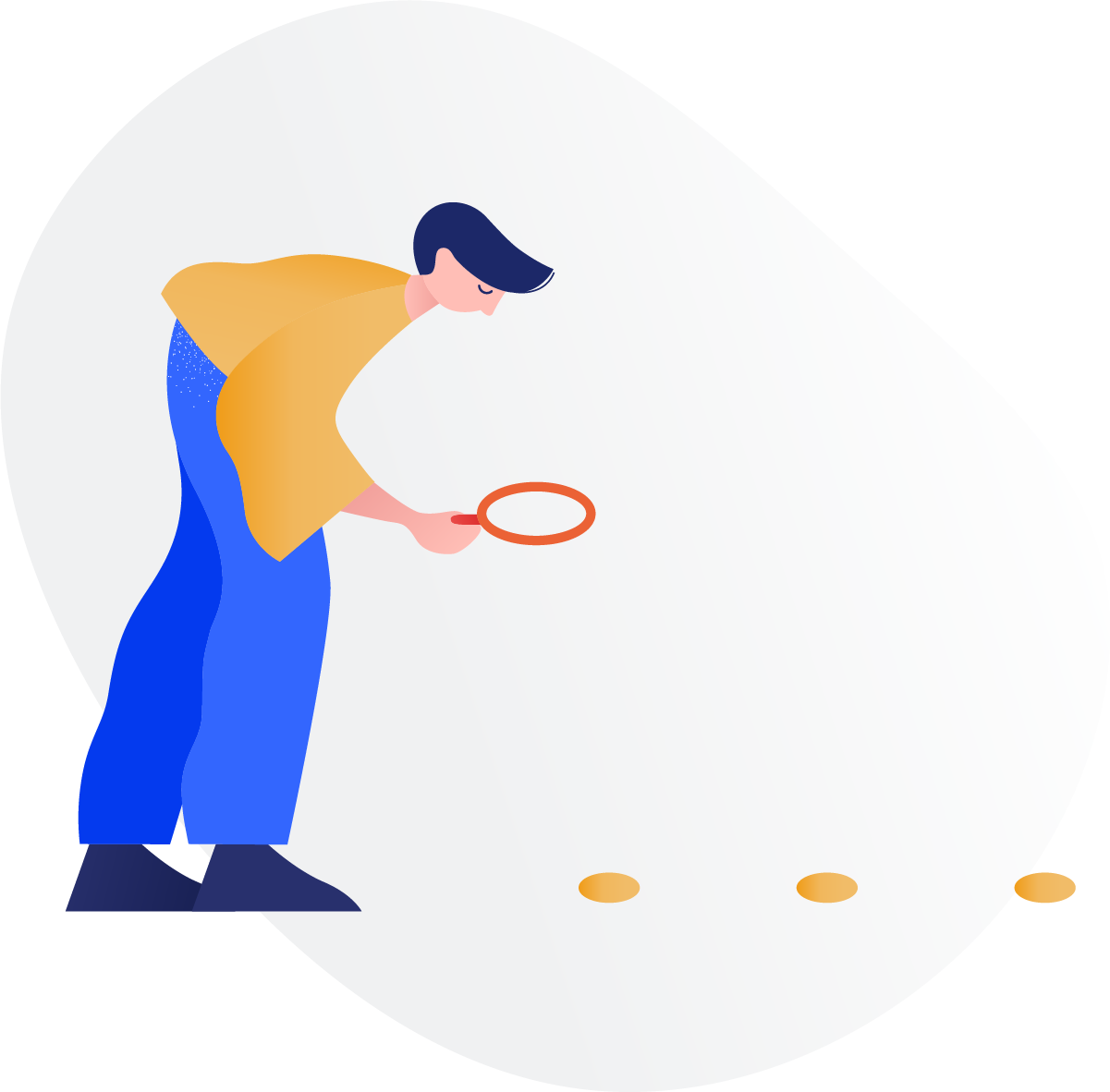
Oops! Something went wrong.
Looks like we were not able to place your request. Kindly try again later.
Generating synthetic gait patterns based on benchmark datasets for controlling prosthetic legs

Journal Article
Generating synthetic gait patterns based on benchmark datasets for controlling prosthetic legs
2023
Request now
and choose the collection method
Overview
Abstract Background Prosthetic legs help individuals with an amputation regain locomotion. Recently, deep neural network (DNN)-based control methods, which take advantage of the end-to-end learning capability of the network, have been proposed. One prominent challenge for these learning-based approaches is obtaining data for the training, particularly for the training of a mid-level controller. In this study, we propose a method for generating synthetic gait patterns (vertical load and lower limb joint angles) using a generative adversarial network (GAN). This approach enables a mid-level controller to execute ambulation modes that are not included in the training datasets. Methods The conditional GAN is trained on benchmark datasets that contain the gait data of individuals without amputation; synthetic gait patterns are generated from the user input. Further, a DNN-based controller for the generation of impedance parameters is trained using the synthetic gait pattern and the corresponding synthetic stiffness and damping coefficients. Results The trained GAN generated synthetic gait patterns with a coefficient of determination of 0.97 and a structural similarity index of 0.94 relative to benchmark data that were not included in the training datasets. We trained a DNN-based controller using the GAN-generated synthetic gait patterns for level-ground walking, standing-to-sitting motion, and sitting-to-standing motion. Four individuals without amputation participated in bypass testing and demonstrated the ambulation modes. The model successfully generated control parameters for the knee and ankle based on thigh angle and vertical load. Conclusions This study demonstrates that synthetic gait patterns can be used to train DNN models for impedance control. We believe a conditional GAN trained on benchmark datasets can provide reliable gait data for ambulation modes that are not included in its training datasets. Thus, designing gait data using a conditional GAN could facilitate the efficient and effective training of controllers for prosthetic legs.
Publisher
BioMed Central Ltd,BioMed Central,BMC
Subject
This website uses cookies to ensure you get the best experience on our website.